Small sample learning method and device, electronic equipment and storage medium
A learning method and small sample technology, applied in the field of machine learning, can solve problems such as limited application, unsatisfactory effect, poor performance of cross-domain tasks, catastrophic forgetting, etc., to achieve the effect of improving efficiency and accuracy, and accelerating learning
- Summary
- Abstract
- Description
- Claims
- Application Information
AI Technical Summary
Problems solved by technology
Method used
Image
Examples
Embodiment 1
[0026] figure 1 It is a flow chart of the small-sample learning method in Embodiment 1 of the present invention, and this embodiment is applicable to the case of performing image classification training on a small-sample data set. The method can be implemented by a small-sample learning device, which can be implemented in the form of software and / or hardware, and can be configured in an electronic device. For example, the electronic device can be a background server and other devices with communication and computing capabilities. like figure 1 As shown, the method specifically includes:
[0027] Step 101: Encode the image training sample set according to the image representation model to obtain an image matrix composed of image vector representations of each image training sample.
[0028] Wherein, the image representation model is used to learn and extract features in the image. Exemplarily, the image representation model may be an encoder composed of a convolutional neural...
Embodiment 2
[0084] image 3 It is a flow chart of the small sample learning method in Embodiment 2 of the present invention. Embodiment 2 is further optimized on the basis of Embodiment 1. After obtaining the trained image representation model and label preprocessing model, complete data The concentrated image test sample set is tested, wherein the image test sample set is a subset of the complete data set. Such as image 3 As shown, the test method includes:
[0085] Step 301. Divide the image test sample set into a support set and a query set; wherein, the support set includes the label of each support image test sample.
[0086] Among them, the query set refers to the image sample set that needs to predict the image classification information; the support set refers to the image sample set that provides label information to provide prediction reference for the image samples to be classified in the query.
[0087] In order to ensure the accuracy of the test results, the image test sa...
Embodiment 3
[0099] Figure 4 It is a schematic structural diagram of a small-sample learning device in Embodiment 3 of the present invention. This embodiment is applicable to the case of performing image classification training on a small-sample data set. Such as Figure 4 As shown, the device includes:
[0100] An image encoding module 410, configured to encode the image training sample set according to the image representation model, to obtain an image matrix composed of image vector representations of each image training sample;
[0101] The label encoding module 420 is used to encode the labels of the image training sample set according to the label preprocessing model to obtain a label matrix composed of label vector representations of each image training sample label;
[0102] A model optimization module 430, configured to perform backpropagation according to the loss values of the image matrix and the label matrix, so as to optimize the parameters of the image representation mo...
PUM
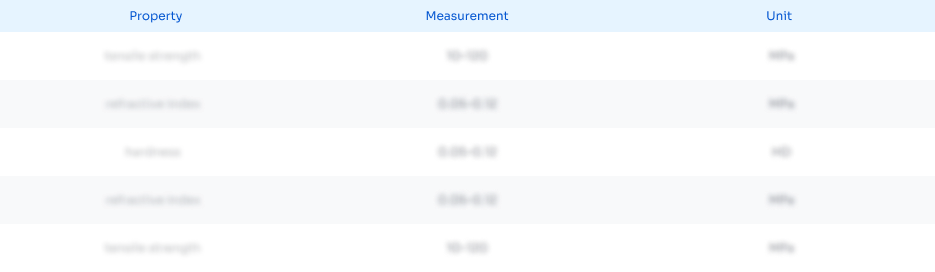
Abstract
Description
Claims
Application Information
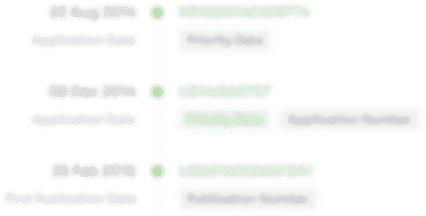
- R&D Engineer
- R&D Manager
- IP Professional
- Industry Leading Data Capabilities
- Powerful AI technology
- Patent DNA Extraction
Browse by: Latest US Patents, China's latest patents, Technical Efficacy Thesaurus, Application Domain, Technology Topic, Popular Technical Reports.
© 2024 PatSnap. All rights reserved.Legal|Privacy policy|Modern Slavery Act Transparency Statement|Sitemap|About US| Contact US: help@patsnap.com