Method for predicting tight oil fracturing range based on DL model of physical constraint
A model prediction, tight oil technology, applied in the field of tight oil fracturing, can solve the problems of not guaranteeing the satisfaction of basic physical laws, time-consuming, high cost, etc.
- Summary
- Abstract
- Description
- Claims
- Application Information
AI Technical Summary
Problems solved by technology
Method used
Image
Examples
Embodiment 1
[0034] The first step is to set the preconditions and assumptions for the problem to be studied. The research area includes the matrix area and the fracturing stimulation area. It is assumed that the strata studied are horizontal, homogeneous, and isotropic; the oil-water flooding model is assumed, and the fluid is single-phase, homogeneous, and weakly compressible Newtonian fluid; the seepage process is assumed Medium is isothermal, without any special physical and chemical phenomena. The length of the horizontal well is set to 1500m, the radius of the stimulated area is set to 250m, and the outside of the stimulated area is the matrix area, and its radius is set to 50m. The density of oil is 860kg / m 3 , the dynamic viscosity of the oil is set to 1.27*10 -3 Pa·s, the original formation pressure is 25MPa, the bottomhole flowing pressure is 15MPa, the initial porosity is 0.1, and the rock compressibility coefficient is -8*10 -4 Pa -1 , considering the 50-day flow field chan...
PUM
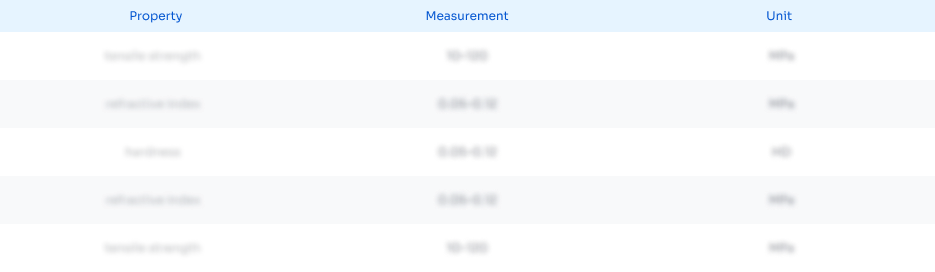
Abstract
Description
Claims
Application Information
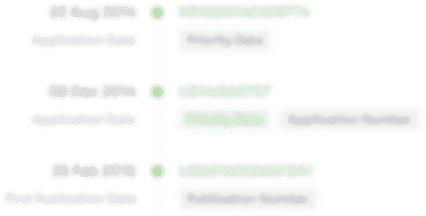
- R&D Engineer
- R&D Manager
- IP Professional
- Industry Leading Data Capabilities
- Powerful AI technology
- Patent DNA Extraction
Browse by: Latest US Patents, China's latest patents, Technical Efficacy Thesaurus, Application Domain, Technology Topic.
© 2024 PatSnap. All rights reserved.Legal|Privacy policy|Modern Slavery Act Transparency Statement|Sitemap