Ship weld defect detection method based on deep convolutional neural network model
A neural network model and deep convolution technology, applied in the field of pattern recognition, can solve problems such as affecting model learning, complex structure, and a large amount of model storage space.
- Summary
- Abstract
- Description
- Claims
- Application Information
AI Technical Summary
Problems solved by technology
Method used
Image
Examples
Embodiment Construction
[0075] In order to make the purpose and technical solutions of the embodiments of the present invention more clear, the technical solutions of the embodiments of the present invention will be clearly and completely described below in conjunction with the drawings of the embodiments of the present invention. Apparently, the described embodiments are some, not all, embodiments of the present invention. Based on the described embodiments of the present invention, all other embodiments obtained by persons of ordinary skill in the art without creative efforts shall fall within the protection scope of the present invention.
[0076] like figure 1 As shown, a ship weld defect detection method based on a deep convolutional neural network model adopts the following steps:
[0077] (1) Obtain ship X-ray weld image collection;
[0078] (2) Processing unbalanced data sets based on the M-SMOTE algorithm of the most distance;
[0079] (3) Construct a deep convolutional neural network mod...
PUM
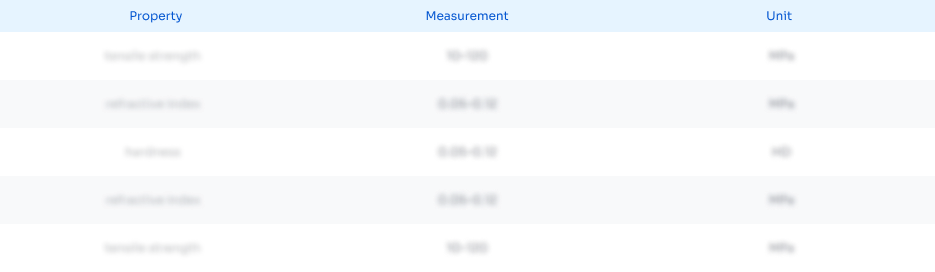
Abstract
Description
Claims
Application Information
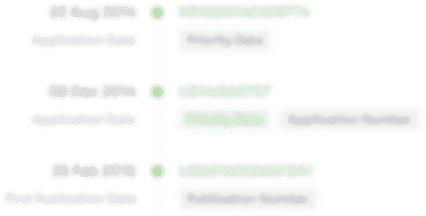
- R&D Engineer
- R&D Manager
- IP Professional
- Industry Leading Data Capabilities
- Powerful AI technology
- Patent DNA Extraction
Browse by: Latest US Patents, China's latest patents, Technical Efficacy Thesaurus, Application Domain, Technology Topic, Popular Technical Reports.
© 2024 PatSnap. All rights reserved.Legal|Privacy policy|Modern Slavery Act Transparency Statement|Sitemap|About US| Contact US: help@patsnap.com