Deep learning method for predicting prognosis risk of cancer patient based on multi-omics data
A technology of omics data and deep learning, applied in machine learning, medical informatics, informatics, etc., can solve problems such as inability to solve target data sets and low accuracy
- Summary
- Abstract
- Description
- Claims
- Application Information
AI Technical Summary
Problems solved by technology
Method used
Image
Examples
Embodiment 1
[0044] Such as figure 1 As shown, a deep learning method for predicting the prognosis risk of cancer patients based on multi-omics data is used to predict the prognosis risk of cancer patients, including the following steps:
[0045] S1: Obtain clinical data Y and corresponding multi-omics expression data X of target cancer patients from existing public data sets (such as TCGA, GEO);
[0046] In a specific embodiment, 14 TCGA data sets (BRCA, CESC, COAD, ESCA, HNSC, KIRC, LGG, LIHC, LUAD, LUSC, MESO, PAAD, SRAC and SKCM) were used for pre-training, and bladder cancer ( BLCA) data as the target cancer.
[0047] Among them, multi-omics data includes mRNA expression, miRNA expression, DNA methylation information and copy number variation information of bladder cancer patients. mRNA data is RNA sequencing data generated by UNC Illumina HiSeq_RNASeq V2. miRNA is the miRNA sequencing data obtained by BCGSC Illumina HiSeq miRNASeq. DNA methylation data was generated by USCHumanMe...
PUM
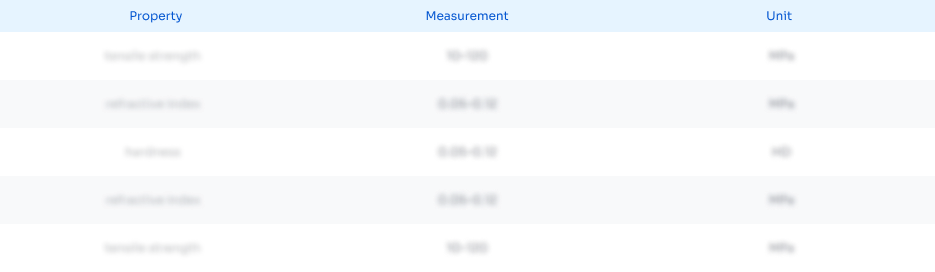
Abstract
Description
Claims
Application Information
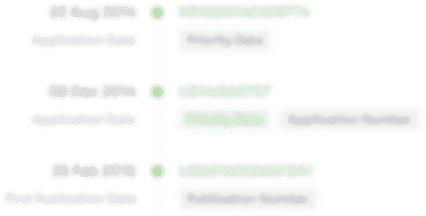
- R&D
- Intellectual Property
- Life Sciences
- Materials
- Tech Scout
- Unparalleled Data Quality
- Higher Quality Content
- 60% Fewer Hallucinations
Browse by: Latest US Patents, China's latest patents, Technical Efficacy Thesaurus, Application Domain, Technology Topic, Popular Technical Reports.
© 2025 PatSnap. All rights reserved.Legal|Privacy policy|Modern Slavery Act Transparency Statement|Sitemap|About US| Contact US: help@patsnap.com