Semantic mapping method based on track alignment
A technology of semantic mapping and trajectory, applied in the field of semantic mapping, can solve problems such as difficult extraction of semantic information and poor accuracy of visual SLAM mapping, achieve good flexibility and scalability, facilitate application, and solve inconsistent trajectory sequences Effect
- Summary
- Abstract
- Description
- Claims
- Application Information
AI Technical Summary
Problems solved by technology
Method used
Image
Examples
Embodiment Construction
[0047] The technical solutions in the embodiments of the present invention will be clearly and completely described below in conjunction with the embodiments of the present invention. Apparently, the described embodiments are only some of the embodiments of the present invention, not all of them. Based on the embodiments of the present invention, all other embodiments obtained by persons of ordinary skill in the art without making creative efforts belong to the protection scope of the present invention.
[0048] The embodiment of the present invention provides a semantic mapping method based on trajectory alignment. Aiming at the problem of traditional SLAM development content coupling, the present invention provides a SLAM development framework based on four levels. On this basis, a multi-sensor based Fusion semantic SLAM algorithm, such as figure 1 and figure 2 shown, including the following steps:
[0049] A1, use the monocular camera and the sweeping robot to scan and c...
PUM
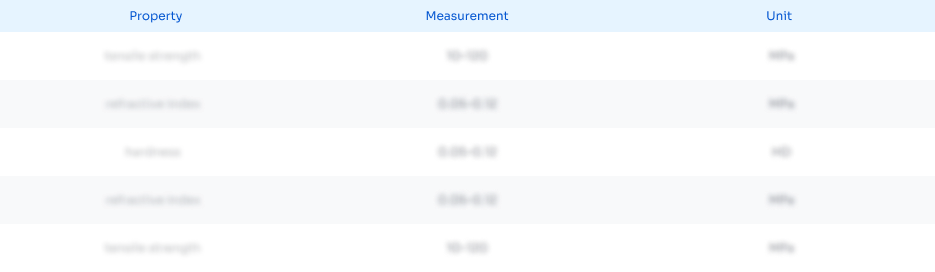
Abstract
Description
Claims
Application Information
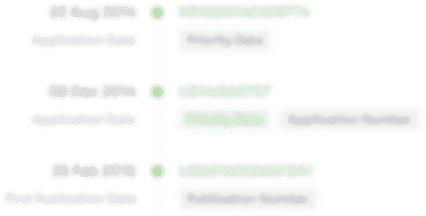
- R&D Engineer
- R&D Manager
- IP Professional
- Industry Leading Data Capabilities
- Powerful AI technology
- Patent DNA Extraction
Browse by: Latest US Patents, China's latest patents, Technical Efficacy Thesaurus, Application Domain, Technology Topic, Popular Technical Reports.
© 2024 PatSnap. All rights reserved.Legal|Privacy policy|Modern Slavery Act Transparency Statement|Sitemap|About US| Contact US: help@patsnap.com