CNN-GRU hierarchical neural network-based network intrusion detection method
A technology of network intrusion detection and neural network, which is applied in the field of network intrusion detection based on CNN-GRU layered neural network, can solve the problems of accuracy bottleneck, unapplicable features, difficult problems, etc., and achieve accuracy improvement and high prediction accuracy Effect
- Summary
- Abstract
- Description
- Claims
- Application Information
AI Technical Summary
Problems solved by technology
Method used
Image
Examples
specific Embodiment
[0061] The CICIDS2017 dataset is used to test the model. The advantage of this dataset is that it has richer traffic types and relatively new data release time, which is more in line with the actual situation of the current network. The dataset comes from an attack scenario devised by the researchers. All data collected on the first day was normal network traffic. Over the next four days, the network was attacked and traffic information was recorded. The end result is stored in a PCAP file, which includes all traffic flagged as normal network traffic and various network attacks. Considering the reliability of the training results, we selected the top ten attack traffic and normal traffic as our training set and test set, ensuring that each type contains at least two thousand traffic data. Given that the labels given in the dataset do not meet the actual needs, we re-added the labels to the traffic data to meet the training requirements. After certain processing, the number ...
PUM
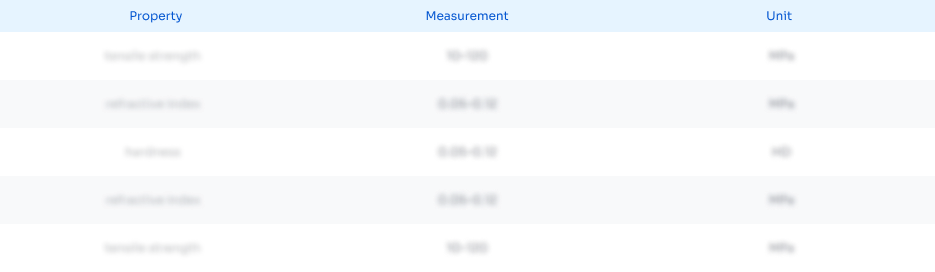
Abstract
Description
Claims
Application Information
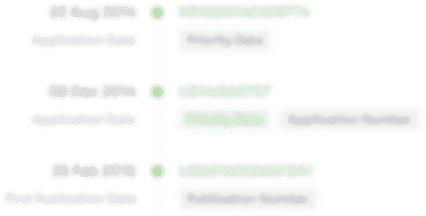
- R&D
- Intellectual Property
- Life Sciences
- Materials
- Tech Scout
- Unparalleled Data Quality
- Higher Quality Content
- 60% Fewer Hallucinations
Browse by: Latest US Patents, China's latest patents, Technical Efficacy Thesaurus, Application Domain, Technology Topic, Popular Technical Reports.
© 2025 PatSnap. All rights reserved.Legal|Privacy policy|Modern Slavery Act Transparency Statement|Sitemap|About US| Contact US: help@patsnap.com