Cancer gene classification method and device based on two-stage depth feature selection and storage medium
A technology of deep features and classification methods, applied in neural learning methods, instruments, biological neural network models, etc., can solve the problems of large number of selected features and low classification accuracy, so as to avoid important genes from being selected, improve classification accuracy, Highly identifiable effect
- Summary
- Abstract
- Description
- Claims
- Application Information
AI Technical Summary
Problems solved by technology
Method used
Image
Examples
Embodiment 1
[0072] A cancer gene classification method based on two-stage deep feature selection, through two-stage deep feature selection to improve the accuracy of cancer classification, such as figure 1 shown, including the following steps:
[0073] A. Training cancer gene classification model
[0074] (1) Obtain training data
[0075] The first stage: Integrate three feature selection algorithms for comprehensive feature selection to obtain feature subsets; this ensures that the final selected feature subsets are small and precise;
[0076] The second stage: using an unsupervised neural network to obtain the best representation of a subset of features; improves the final classification accuracy.
[0077] (2) Divide the best representation of the feature subset into a training set and a test set, and input it into the cancer gene classification model for training;
[0078] B. Cancer gene classification
[0079] Preprocess the cancer gene data to be detected and input it into the trai...
Embodiment 2
[0081] According to a kind of cancer gene classification method based on two-stage deep feature selection described in Example 1, the difference is:
[0082] In step B, the process of preprocessing the detected cancer gene data is as follows: After removing the null value and non-numeric data in the cancer gene data to be detected, the best representation of the feature subset is obtained through the first and second stages of processing, Feed a subset of features into a trained cancer gene classification model.
[0083] The present invention realizes feature selection in consideration of various aspects by using an integrated feature selection method; and extracts the best representation of features by using a non-supervised neural network to obtain cleaner gene features and improve classification accuracy.
Embodiment 3
[0085] According to a kind of cancer gene classification method based on two-stage deep feature selection described in Example 1, such as figure 1 As shown, the difference is:
[0086] Based on the boosting integrated feature selection method, three feature selection algorithms are integrated to achieve comprehensive feature selection. The three feature selection algorithms include analysis of variance (ANOVA), RReliefF algorithm and random forest algorithm (RF); the implementation process of comprehensive feature selection is as follows:
[0087] (1) Perform feature selection on the original data through variance analysis and RReliefF algorithm, and obtain candidate feature subsets according to two internal operations;
[0088] (2) Use the random forest algorithm to sort the candidate feature subsets according to the feature importance, and select the required feature subsets.
[0089] These three methods take into account the characteristics of gene features themselves, the...
PUM
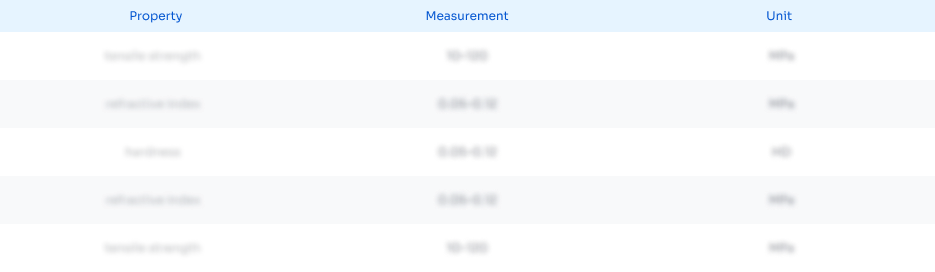
Abstract
Description
Claims
Application Information
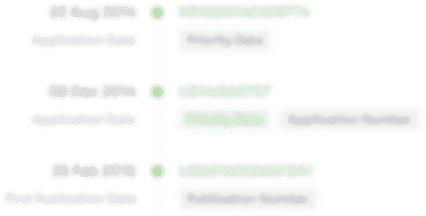
- R&D
- Intellectual Property
- Life Sciences
- Materials
- Tech Scout
- Unparalleled Data Quality
- Higher Quality Content
- 60% Fewer Hallucinations
Browse by: Latest US Patents, China's latest patents, Technical Efficacy Thesaurus, Application Domain, Technology Topic, Popular Technical Reports.
© 2025 PatSnap. All rights reserved.Legal|Privacy policy|Modern Slavery Act Transparency Statement|Sitemap|About US| Contact US: help@patsnap.com