Road disaster remote sensing intelligent detection method based on deep learning
A technology of intelligent detection and deep learning, applied in the direction of neural learning methods, instruments, biological neural network models, etc., can solve the problems of road disaster detection accuracy dependence, road extraction accuracy, long detection time, and low degree of automation, etc., to achieve narrow detection Range, noise removal detection, effect of narrowing the range
- Summary
- Abstract
- Description
- Claims
- Application Information
AI Technical Summary
Problems solved by technology
Method used
Image
Examples
Embodiment 1
[0046] Such as figure 1 As shown, in order to solve the problems of low automaticity and human intervention in traditional methods, the present invention proposes an intelligent road disaster detection method based on deep learning, using the deep learning YOLOV3 model with fast speed and high detection accuracy of small targets as the The core network performs road disaster target detection. Aiming at the problem that the detection accuracy of road disasters depends too much on the accuracy of road extraction and disaster extraction, the present invention uses contextual semantic knowledge and combines the background characteristics of road disasters after the earthquake to construct road disaster target samples and directly locate road disaster targets themselves, which solves the problem of road disasters in the past. The problem of high dependence on the accuracy of disaster extraction enables road disaster detection with a higher degree of automation and improves detectio...
PUM
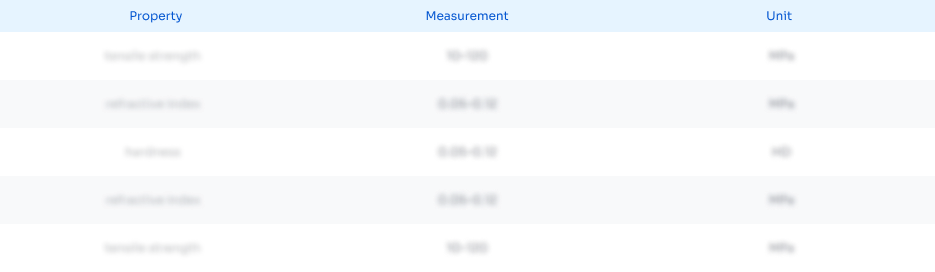
Abstract
Description
Claims
Application Information
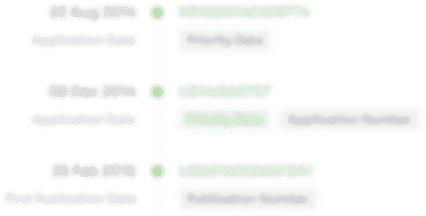
- Generate Ideas
- Intellectual Property
- Life Sciences
- Materials
- Tech Scout
- Unparalleled Data Quality
- Higher Quality Content
- 60% Fewer Hallucinations
Browse by: Latest US Patents, China's latest patents, Technical Efficacy Thesaurus, Application Domain, Technology Topic, Popular Technical Reports.
© 2025 PatSnap. All rights reserved.Legal|Privacy policy|Modern Slavery Act Transparency Statement|Sitemap|About US| Contact US: help@patsnap.com