Sketch-based interactive iteration type virtual shoeprint image generation method
An image generation and shoe print technology, which is applied in image enhancement, image analysis, image data processing, etc., can solve problems such as difficult extraction of shoe print images, only applicable models, and smooth generated images, so as to improve the loss function and improve the fitting Ability, the effect of improving the ability to judge
- Summary
- Abstract
- Description
- Claims
- Application Information
AI Technical Summary
Problems solved by technology
Method used
Image
Examples
Embodiment 1
[0097] figure 2 It is a multi-level fusion of hole residuals and fully convolutional shoe print image generation network, in which the solid line part is the multi-scale hole convolution fusion module, and the dotted line part is the hole convolution residual multi-level fusion module, and the solid line represents the convolution. K represents the span, d represents the dilation rate, Conv3 represents the convolution kernel size is 3×3, and the Conv number represents the number of convolution kernels. For example, Conv3, 512 in the figure, K=1, d=2, means that the convolution kernel size of the convolution layer is 3×3, the number of convolution kernels is 512, the span is 1, and the expansion coefficient is 2. The dotted connection line represents Concat, the dotted connection line represents deconvolution, and ConvT3 represents deconvolution with a convolution kernel size of 3×3.
Embodiment 2
[0099] image 3 It is a fully convolutional deep and shallow layer feature fusion shoeprint image discrimination network, where the solid line part represents the deep and shallow layer expansion convolution feature fusion module, and the dotted line part is the span convolution downsampling module. The solid connection line represents convolution, K represents the span, d represents the dilation rate, Conv3 represents the convolution kernel size is 3×3, and the Conv number represents the number of convolution kernels. For example, Conv3 in the figure, 256, K=1, d=2, means that the convolution kernel size of the convolution layer is 3×3, the number of convolution kernels is 256, the span is 1, and the expansion coefficient is 2. Dotted lines represent concatenation (Concat).
PUM
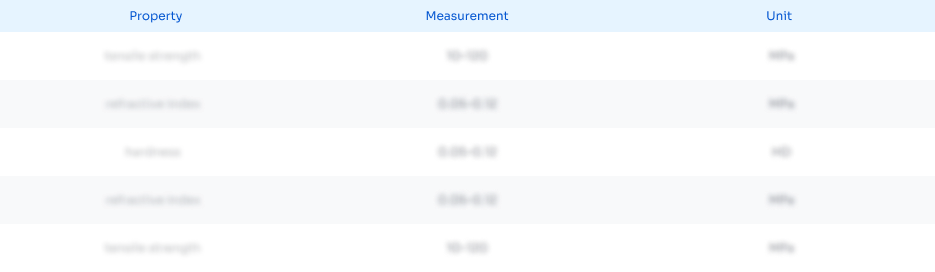
Abstract
Description
Claims
Application Information
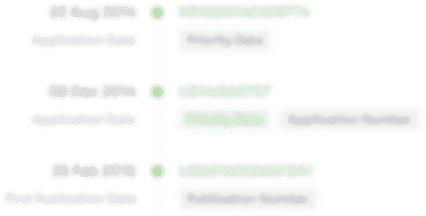
- R&D
- Intellectual Property
- Life Sciences
- Materials
- Tech Scout
- Unparalleled Data Quality
- Higher Quality Content
- 60% Fewer Hallucinations
Browse by: Latest US Patents, China's latest patents, Technical Efficacy Thesaurus, Application Domain, Technology Topic, Popular Technical Reports.
© 2025 PatSnap. All rights reserved.Legal|Privacy policy|Modern Slavery Act Transparency Statement|Sitemap|About US| Contact US: help@patsnap.com