A kind of abnormal behavior detection method and system
A detection method and behavior technology, applied in the field of deep learning, can solve the problems of abnormal behavior recognition accuracy, poor operation convenience, failure to meet abnormal behavior detection requirements, and low accuracy of abnormal behavior recognition, so as to reduce network transmission pressure , enhance the classification accuracy, and reduce the effect of manual participation
- Summary
- Abstract
- Description
- Claims
- Application Information
AI Technical Summary
Problems solved by technology
Method used
Image
Examples
Embodiment Construction
[0037] Such as figure 1 As shown, the architecture of Embodiment 1 of the present invention consists of three parts: (1) IoT device layer: IoT devices such as hemispheres and global cameras record real-time video sequences and transmit them to edge servers for abnormal behavior detection. (2) Edge server: The edge server identifies the video sequence that appears in the IoT device layer, if it is in the known behavior category in the current edge server system. If it is identified as an abnormal behavior, upload the behavior data to the cloud and wait for the cloud to reply. The edge server saves the newly added behavior categories sent back from the cloud to the edge server system, and performs abnormal behavior detection on the current video sequence again to complete behavior recognition. (3) Cloud: Since the cloud has global knowledge of distributed edge servers and more powerful computing capabilities, it can help edge servers detect abnormal behavior category data. Spe...
PUM
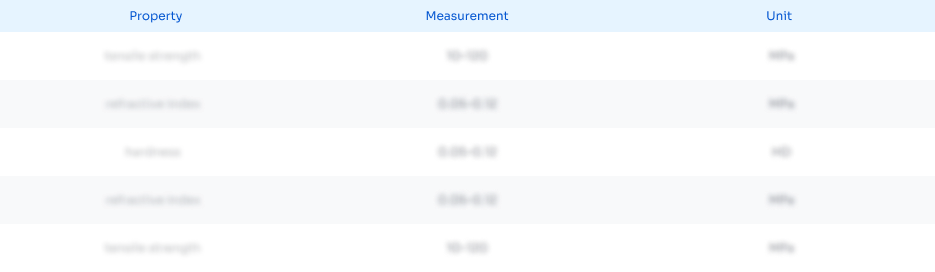
Abstract
Description
Claims
Application Information
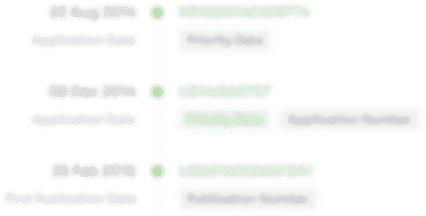
- R&D Engineer
- R&D Manager
- IP Professional
- Industry Leading Data Capabilities
- Powerful AI technology
- Patent DNA Extraction
Browse by: Latest US Patents, China's latest patents, Technical Efficacy Thesaurus, Application Domain, Technology Topic, Popular Technical Reports.
© 2024 PatSnap. All rights reserved.Legal|Privacy policy|Modern Slavery Act Transparency Statement|Sitemap|About US| Contact US: help@patsnap.com