Deep learning-based sea wave height prediction method and application thereof
A technology of deep learning and prediction methods, applied in neural learning methods, biological neural network models, design optimization/simulation, etc., can solve problems such as poor adaptability, large data requirements and processing volume, and inability to fully exploit the relationship between wave height data, etc. Achieve strong generalization performance and high prediction accuracy
- Summary
- Abstract
- Description
- Claims
- Application Information
AI Technical Summary
Problems solved by technology
Method used
Image
Examples
Embodiment 1
[0034] A method for predicting the height of sea waves based on deep learning, applied to electronic equipment, the steps are as follows (specifically as figure 1 shown):
[0035] (1) Perform data preprocessing on the wave data information including wind speed, wind direction and other variables and wave height values, specifically: after analyzing the wave data information to confirm the abnormal value, calculate the average value of the abnormal value and use The average value replaces the abnormal value, and finally the data is normalized;
[0036] (2) Input the wave data information after data preprocessing into the AM-LSTM model and the CatBoost model to obtain output P1 and P2;
[0037] (3) According to the following formula, P1 and P2 are reconstructed to obtain the predicted sequence P;
[0038] P=q2*P1+q1*P2,
[0039]
[0040]
[0041] Among them, w1 refers to the mean value of MAE, RMSE and MAPE output by the AM-LSTM model, and w2 refers to the mean value of...
Embodiment 2
[0049] An electronic device, including one or more processors, one or more memories, one or more programs, and a parameter acquisition device for acquiring ocean wave data information;
[0050] One or more programs are stored in the memory, and when the one or more programs are executed by the processor, the electronic device executes the sea wave height prediction method as described in Embodiment 1.
PUM
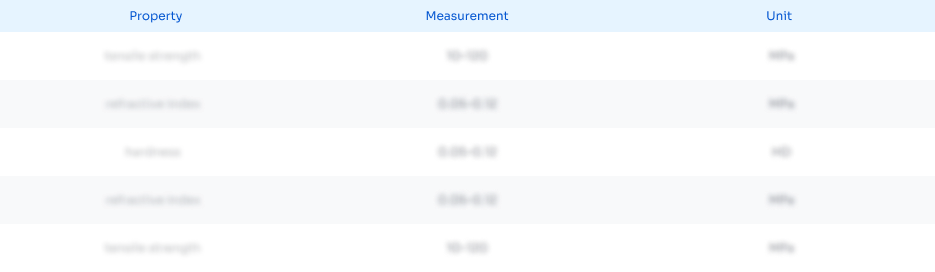
Abstract
Description
Claims
Application Information
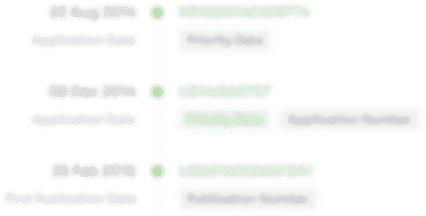
- R&D
- Intellectual Property
- Life Sciences
- Materials
- Tech Scout
- Unparalleled Data Quality
- Higher Quality Content
- 60% Fewer Hallucinations
Browse by: Latest US Patents, China's latest patents, Technical Efficacy Thesaurus, Application Domain, Technology Topic, Popular Technical Reports.
© 2025 PatSnap. All rights reserved.Legal|Privacy policy|Modern Slavery Act Transparency Statement|Sitemap|About US| Contact US: help@patsnap.com