Chip defect image classification method based on ResNet network
A chip defect and classification method technology, applied in the field of deep learning, can solve problems such as high false detection rate, poor real-time performance, and inability to describe quantitatively, and achieve the effect of improving performance and enhancing classification accuracy
- Summary
- Abstract
- Description
- Claims
- Application Information
AI Technical Summary
Problems solved by technology
Method used
Image
Examples
Embodiment 1
[0042] A chip defect image classification method based on the ResNet network, which is classified through the ResNet network, including
[0043] Divide the obtained sample data into 90% training set, 5% validation set and 5% test set;
[0044] Sample pretreatment;
[0045] 90% of the training set and 5% of the verification set in the processed sample images are used to train the network model that has been built;
[0046] The trained network model is used as the test model, and the remaining 5% of the test set is used in the test network, and finally the classification result is output through the activation function.
[0047] The chip is a high-speed laser chip.
[0048] The sample pretreatment includes
[0049] (1) Image segmentation
[0050] The size of the original image is large. In order to process the entire image, it takes a lot of computing work and time to extract the interested part of the marked defect in the chip defect image into an image block with a small s...
PUM
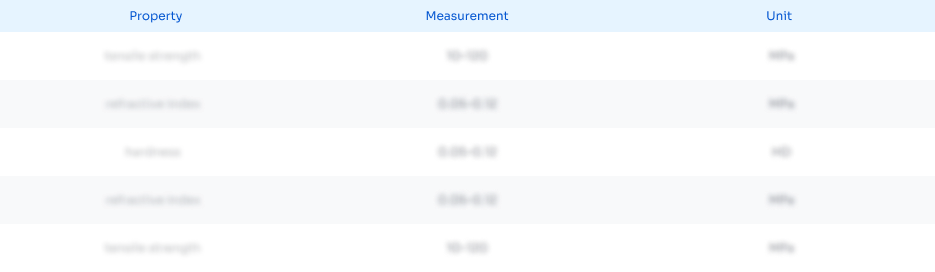
Abstract
Description
Claims
Application Information
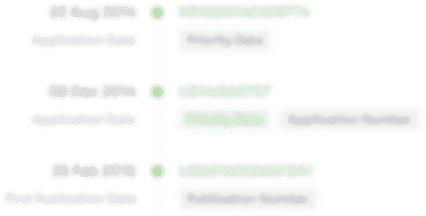
- R&D Engineer
- R&D Manager
- IP Professional
- Industry Leading Data Capabilities
- Powerful AI technology
- Patent DNA Extraction
Browse by: Latest US Patents, China's latest patents, Technical Efficacy Thesaurus, Application Domain, Technology Topic, Popular Technical Reports.
© 2024 PatSnap. All rights reserved.Legal|Privacy policy|Modern Slavery Act Transparency Statement|Sitemap|About US| Contact US: help@patsnap.com