Depth map confidence estimation method based on convolutional neural network
A convolutional neural network and confidence level technology, applied in the field of quality assessment of depth maps, can solve problems such as low precision and inability to make full use of multi-modal data, and achieve an effect that is beneficial to post-processing
- Summary
- Abstract
- Description
- Claims
- Application Information
AI Technical Summary
Problems solved by technology
Method used
Image
Examples
Embodiment Construction
[0034] Exemplary embodiments of the present disclosure will be described in more detail below with reference to the accompanying drawings. Although exemplary embodiments of the present disclosure are shown in the drawings, it should be understood that the present disclosure may be embodied in various forms and should not be limited by the embodiments set forth herein. Rather, these embodiments are provided for more thorough understanding of the present disclosure and to fully convey the scope of the present disclosure to those skilled in the art.
[0035] The present invention proposes a depth map confidence estimation network based on multi-purpose stereo matching, which preliminarily solves this problem. The truncated symbol distance function map (tsdf) and color map generated by the multi-purpose stereo matching algorithm are analyzed by using the U-Net structure. Feature extraction is performed with the normal map, and the confidence of the depth map is predicted from the ...
PUM
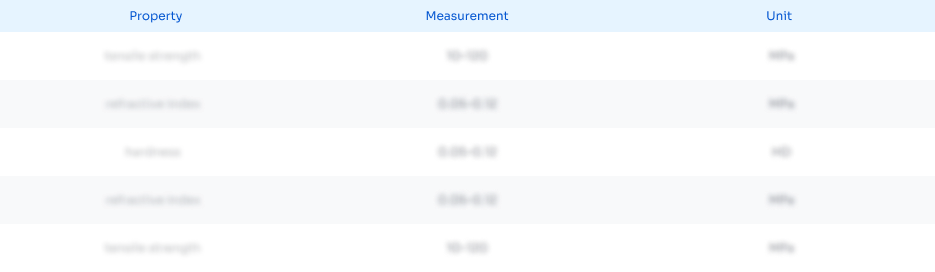
Abstract
Description
Claims
Application Information
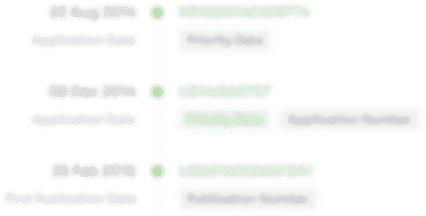
- R&D
- Intellectual Property
- Life Sciences
- Materials
- Tech Scout
- Unparalleled Data Quality
- Higher Quality Content
- 60% Fewer Hallucinations
Browse by: Latest US Patents, China's latest patents, Technical Efficacy Thesaurus, Application Domain, Technology Topic, Popular Technical Reports.
© 2025 PatSnap. All rights reserved.Legal|Privacy policy|Modern Slavery Act Transparency Statement|Sitemap|About US| Contact US: help@patsnap.com