Graph convolutional neural network action recognition method based on attention mechanism
A convolutional neural network and action recognition technology, applied in the field of graph convolutional neural network action recognition based on attention mechanism, can solve the problems of ignoring skeleton time information, high computational complexity of graph learning, unable to capture the spatial relationship of nodes, etc. Achieve the effect of reducing information processing redundancy and high recognition rate
- Summary
- Abstract
- Description
- Claims
- Application Information
AI Technical Summary
Problems solved by technology
Method used
Image
Examples
Embodiment Construction
[0057] The present invention will be further described below in conjunction with accompanying drawing.
[0058] In the present invention, the flow chart of the graph convolutional neural network action recognition method based on the attention mechanism is as attached figure 1 As shown, the implementation steps are as follows:
[0059] Step 1. Use the residual attention network to mark the N attention joints with the highest action participation. N can be 16, or other values can be set according to the actual situation:
[0060] A residual attention network is used to extract attention joints in 3D skeleton information. The core part of the residual attention network is a multi-layer overlay attention module. Each attention module includes a mask branch and a trunk branch. The main branch performs feature processing and can use any network model. The residual attention network takes as input the raw RGB image corresponding to the skeleton information to generate attentio...
PUM
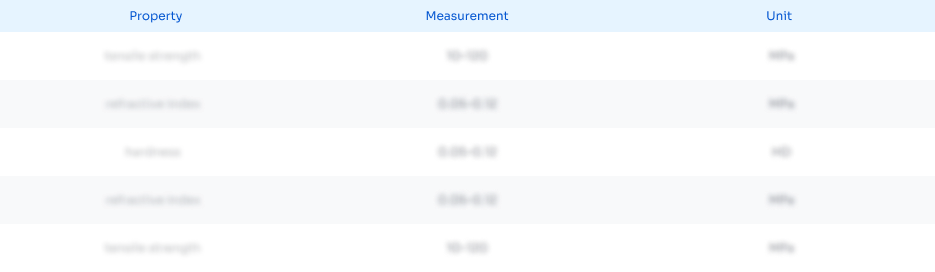
Abstract
Description
Claims
Application Information
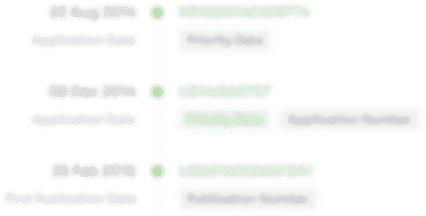
- R&D Engineer
- R&D Manager
- IP Professional
- Industry Leading Data Capabilities
- Powerful AI technology
- Patent DNA Extraction
Browse by: Latest US Patents, China's latest patents, Technical Efficacy Thesaurus, Application Domain, Technology Topic, Popular Technical Reports.
© 2024 PatSnap. All rights reserved.Legal|Privacy policy|Modern Slavery Act Transparency Statement|Sitemap|About US| Contact US: help@patsnap.com