Entity recognition method based on semi-supervised learning and clustering
A technology for rail transit and entity recognition, applied in neural learning methods, text database clustering/classification, biological neural network models, etc. data and other issues, to achieve the effect of improving the extraction speed and accuracy, shortening the processing time, and increasing the query rate
- Summary
- Abstract
- Description
- Claims
- Application Information
AI Technical Summary
Problems solved by technology
Method used
Image
Examples
Embodiment
[0075] The object of the present invention provides a kind of rail transit specification named entity recognition method based on semi-supervised and clustering, concrete frame is as follows figure 1 shown. Experts build ontology databases in the field of rail transit, and manually label part of the data; use word2vec and BERT pre-training models to vectorize labeled entities; secondly, use hierarchical clustering methods to cluster entity word vectors, and entities defined by experts Category proofreading, finalized entity categories; data preprocessing and data training on the training data again, input the generated word vectors into the BiLSTM-CRF algorithm to train the named entity recognition model, and use the Softmax function to iteratively train and optimize the extracted entity features Entity recognition model; set the deep learning model as the server to test the effect of the entity recognition model, input the test data set into the model to output the entity cat...
example
[0130] Entity labeling of the rail transit specification corpus, the specific steps are as follows:
[0131] Step 11.3.1, taking the subway design specification "9.1.6 Stations should be equipped with barrier-free facilities" as an example, the training set output by the BERT model is vectorized, and each word in "Stations should be equipped with barrier-free facilities" is trained Get a 768-dimensional vector, get the initialization vector of each word, and then use the result as the input of the deep learning model.
[0132] In step 11.3.2, using the BiLSTM-CRF algorithm in deep learning, bidirectional LSTM considers both past features and future features, a forward input sequence, and a reverse input sequence to predict the semantics of words in context. For example, after inputting "station", BiLSTM will predict the probability that the next word is "ying", and then input "station should" to predict the probability of the next word "setting", which is a positive inpu...
PUM
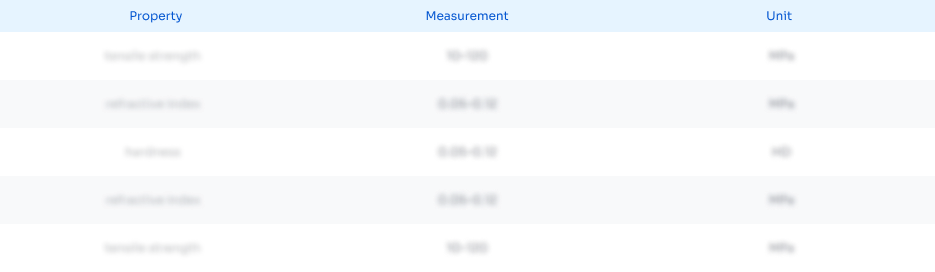
Abstract
Description
Claims
Application Information
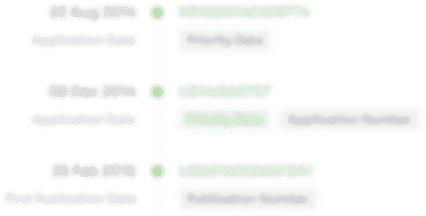
- R&D Engineer
- R&D Manager
- IP Professional
- Industry Leading Data Capabilities
- Powerful AI technology
- Patent DNA Extraction
Browse by: Latest US Patents, China's latest patents, Technical Efficacy Thesaurus, Application Domain, Technology Topic, Popular Technical Reports.
© 2024 PatSnap. All rights reserved.Legal|Privacy policy|Modern Slavery Act Transparency Statement|Sitemap|About US| Contact US: help@patsnap.com