Cross-modal MR image mutual generation method based on cyclic generative adversarial network CycleGAN model
A cross-modal, imaging technology, applied in the field of computer vision, can solve the problems of loss of biological tissue structure information, difficult acquisition of MR images, low image quality, etc. Effect
- Summary
- Abstract
- Description
- Claims
- Application Information
AI Technical Summary
Problems solved by technology
Method used
Image
Examples
Embodiment Construction
[0019] In order to make the technical means, creative features, goals and effects of the present invention easy to understand, the following embodiments will specifically illustrate a cross-modal MR image mutual generation method based on the CycleGAN model of the present invention in conjunction with the accompanying drawings.
[0020]
[0021] This embodiment describes in detail the cross-modal MR image mutual generation method based on the CycleGAN model.
[0022] figure 1 It is a schematic structural diagram of the cycle generation confrontation network CycleGAN model in this embodiment.
[0023] Such as figure 1 As shown, the CycleGAN model includes a generator and a discriminator.
[0024] The generator consists of a generator input layer, a convolutional layer, a residual block, and a deconvolutional layer.
[0025] The input to the generator input layer is the source modality MR image. The input source modality MR image in this embodiment is brain T1 weighted MR ...
PUM
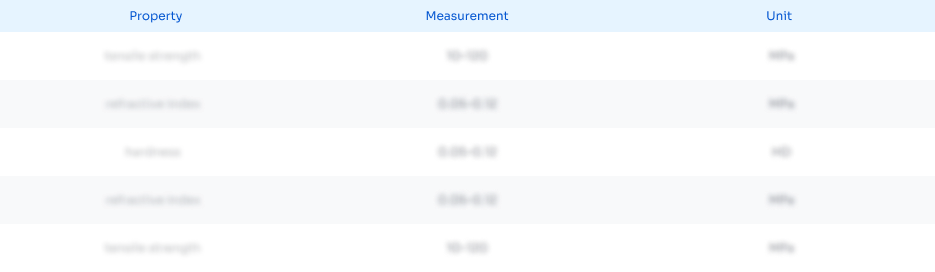
Abstract
Description
Claims
Application Information
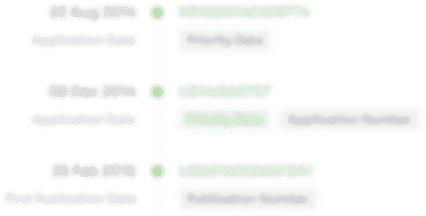
- R&D
- Intellectual Property
- Life Sciences
- Materials
- Tech Scout
- Unparalleled Data Quality
- Higher Quality Content
- 60% Fewer Hallucinations
Browse by: Latest US Patents, China's latest patents, Technical Efficacy Thesaurus, Application Domain, Technology Topic, Popular Technical Reports.
© 2025 PatSnap. All rights reserved.Legal|Privacy policy|Modern Slavery Act Transparency Statement|Sitemap|About US| Contact US: help@patsnap.com