Short-term traffic flow prediction method based on global-local residual combination model
A short-term traffic flow, combined model technology, applied in traffic flow detection, road vehicle traffic control system, traffic control system and other directions, can solve the lack of global consideration, the model prediction accuracy is not high, the model can not adapt to urban road network traffic Stream prediction and other issues to achieve the effect of reducing training errors, improving capture, and improving training accuracy
- Summary
- Abstract
- Description
- Claims
- Application Information
AI Technical Summary
Problems solved by technology
Method used
Image
Examples
Embodiment Construction
[0044] The technical method of the present invention will be further described in detail below in conjunction with the accompanying drawings.
[0045] like Figure 1-3 As shown, a short-term traffic flow prediction method of urban road network based on global-local spatio-temporal residual combination model includes the following steps:
[0046] Step 1) Collect urban road network traffic flow data and transmit it to the traffic big data cluster in real time, perform data preprocessing on the original urban road network traffic flow data to reduce data redundancy, and divide the preprocessed urban road network traffic data according to the latitude and longitude of the road network Streaming data into spatio-temporal raster data;
[0047] In the described step 1, according to the longitude and latitude of the road network, the data after the preprocessing is converted into spatiotemporal raster data, specifically: the urban road network is divided into the network area of I*...
PUM
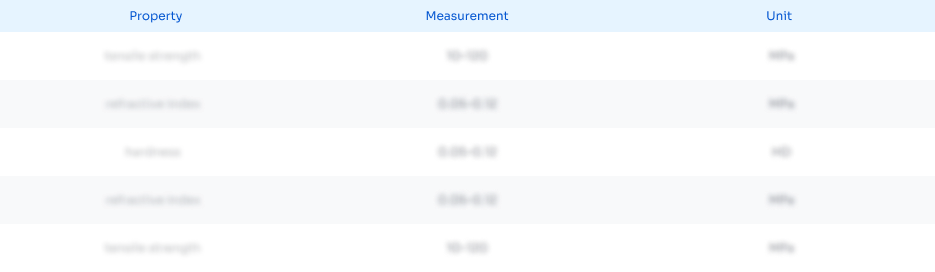
Abstract
Description
Claims
Application Information
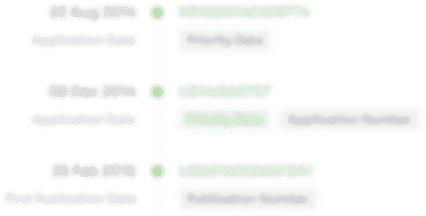
- R&D Engineer
- R&D Manager
- IP Professional
- Industry Leading Data Capabilities
- Powerful AI technology
- Patent DNA Extraction
Browse by: Latest US Patents, China's latest patents, Technical Efficacy Thesaurus, Application Domain, Technology Topic.
© 2024 PatSnap. All rights reserved.Legal|Privacy policy|Modern Slavery Act Transparency Statement|Sitemap