Charging space-time prediction method of mixed isomorphic and heterogeneous depth map neural network
A deep neural network, neural network technology, applied in the field of electric vehicles, can solve the problem that dynamic programming is not suitable for real-time control and so on
- Summary
- Abstract
- Description
- Claims
- Application Information
AI Technical Summary
Problems solved by technology
Method used
Image
Examples
Embodiment Construction
[0059] A charging space-time prediction method of a mixed isomorphic and heterogeneous depth map neural network proposed by the present invention is described in detail in conjunction with the accompanying drawings as follows:
[0060] figure 1 is a schematic diagram of a hybrid isomorphic and heterogeneous deep graph neural network of the method of the present invention. A homogeneous network refers to a network in which all nodes have the same function in the network, that is, one user can exchange basic functions with the next user. For example, in a fixed telephone network, where each node (telephone) performs essentially the same communication function as any other node, and people use phones for the same reasons, telecommunications networks are usually homogeneous (homogeneous) networks.
[0061] A heterogeneous network refers to a network in which nodes are divided into two or more classes by function and utility. For example, on the Honeybook market network, event pl...
PUM
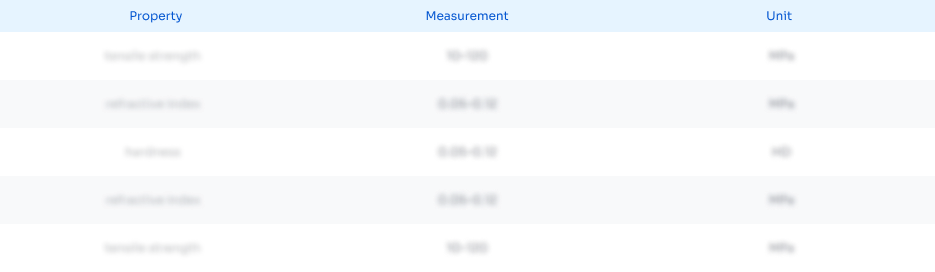
Abstract
Description
Claims
Application Information
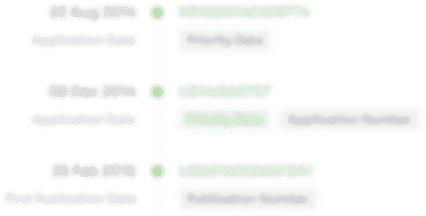
- R&D
- Intellectual Property
- Life Sciences
- Materials
- Tech Scout
- Unparalleled Data Quality
- Higher Quality Content
- 60% Fewer Hallucinations
Browse by: Latest US Patents, China's latest patents, Technical Efficacy Thesaurus, Application Domain, Technology Topic, Popular Technical Reports.
© 2025 PatSnap. All rights reserved.Legal|Privacy policy|Modern Slavery Act Transparency Statement|Sitemap|About US| Contact US: help@patsnap.com