Multi-step daily runoff forecasting method based on meteorological information and deep learning algorithm
A technology of deep learning and meteorological information, applied in the field of multi-step daily runoff forecasting, can solve problems such as insufficient accuracy of runoff forecasting, and achieve the effect of reducing lightning disasters and flood disasters
- Summary
- Abstract
- Description
- Claims
- Application Information
AI Technical Summary
Problems solved by technology
Method used
Image
Examples
Embodiment Construction
[0033] The present invention will be further described below in conjunction with the accompanying drawings and examples of implementation.
[0034] The overall structural framework of the present invention is the GBRT-MIC model. The GBRT-MIC method adopts a three-stage modeling. First, the first stage uses the maximum mutual information coefficient MIC to select the features of the meteorological data set as the model's predictor candidate input, and uses CCF and PACF to analyze the historical lag data of observed runoff and rainfall. Selection is made as the predictor candidate input of the model; in the second stage, data scaling is performed on the selected predictors, and then the data set is divided into training set, validation set and test set. In the third stage, the parameters of the GBRT model are calibrated through the grid search algorithm, and then the prediction and result evaluation are carried out on the test set using the optimal parameters. The specific proc...
PUM
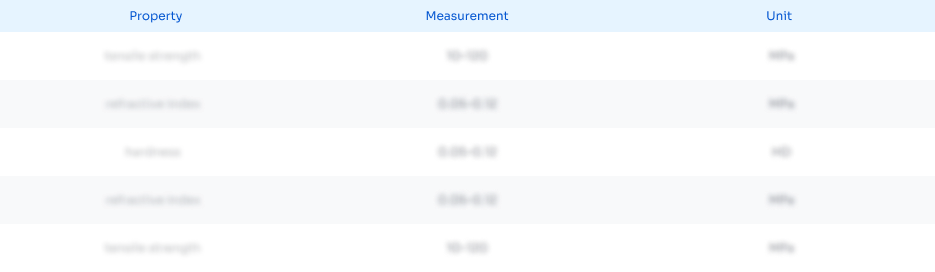
Abstract
Description
Claims
Application Information
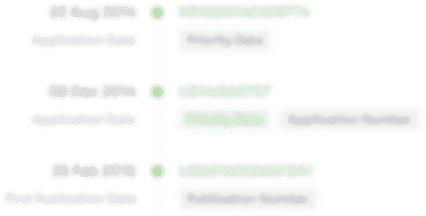
- R&D
- Intellectual Property
- Life Sciences
- Materials
- Tech Scout
- Unparalleled Data Quality
- Higher Quality Content
- 60% Fewer Hallucinations
Browse by: Latest US Patents, China's latest patents, Technical Efficacy Thesaurus, Application Domain, Technology Topic, Popular Technical Reports.
© 2025 PatSnap. All rights reserved.Legal|Privacy policy|Modern Slavery Act Transparency Statement|Sitemap|About US| Contact US: help@patsnap.com