Deep learning defect automatic detection and identification method based on small sample aero-engine blade CT image
An aero-engine and deep learning technology, applied in the field of automatic detection and identification of deep learning defects, can solve the problems of inability to effectively improve the factory quality of turbine blades, complex casting process of turbine blades, high missed detection rate and false detection rate, and achieve high efficiency and intelligence The effect of improving defect detection and recognition performance, detection efficiency and detection accuracy
- Summary
- Abstract
- Description
- Claims
- Application Information
AI Technical Summary
Problems solved by technology
Method used
Image
Examples
Embodiment Construction
[0038]In describing the present invention, it should be understood that the terms "center", "longitudinal", "transverse", "length", "width", "thickness", "upper", "lower", "front", " Orientation indicated by rear, left, right, vertical, horizontal, top, bottom, inside, outside, clockwise, counterclockwise, etc. The positional relationship is based on the orientation or positional relationship shown in the drawings, which is only for the convenience of describing the present invention and simplifying the description, rather than indicating or implying that the referred device or element must have a specific orientation, be constructed and operated in a specific orientation, Therefore, it should not be construed as limiting the invention.
[0039] see Figure 1-Figure 6 , in order to achieve the above object, the technical scheme that the present invention adopts is as follows:
[0040] The present invention is a deep learning defect automatic detection and recognition method ...
PUM
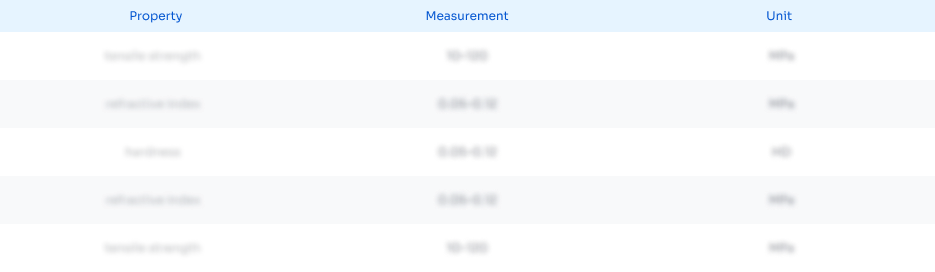
Abstract
Description
Claims
Application Information
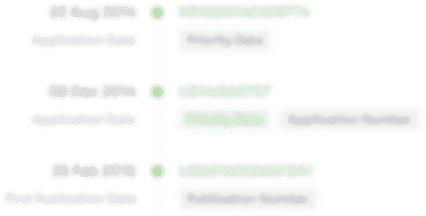
- R&D
- Intellectual Property
- Life Sciences
- Materials
- Tech Scout
- Unparalleled Data Quality
- Higher Quality Content
- 60% Fewer Hallucinations
Browse by: Latest US Patents, China's latest patents, Technical Efficacy Thesaurus, Application Domain, Technology Topic, Popular Technical Reports.
© 2025 PatSnap. All rights reserved.Legal|Privacy policy|Modern Slavery Act Transparency Statement|Sitemap|About US| Contact US: help@patsnap.com