Reactive voltage control method based on multi-time-scale multi-agent deep reinforcement learning
A multi-time scale, multi-agent technology, applied in neural learning methods, reactive power compensation, AC network voltage adjustment and other directions, can solve the problems of slow calculation speed, falling into local optimum and other problems, achieve fast real-time scheduling, reduce voltage and other problems. The effect of bias
- Summary
- Abstract
- Description
- Claims
- Application Information
AI Technical Summary
Problems solved by technology
Method used
Image
Examples
Embodiment Construction
[0074] Inspired by the data-driven method, the applicant's inventor team defined the on-load tap changer (oLTC), capacitor bank (CB) and energy storage (ES) in photovoltaics, wind turbines and loads as intelligent agents. Reinforcement learning methods are applied to reactive power optimization problems, allowing the controller to learn control strategies by interacting with simulation models of similar systems. The action variable of the reactive power regulating equipment interacts with the distribution network environment, and by using the time series in mathematics to describe the interaction process as a process called Markov decision process (markov decision process), the agent can finally realize the external The optimal response to the environment, so as to obtain the maximum return value. Use the neural network method to analyze and fit the strategy function and action value function of each agent. The training process does not depend on the predicted data results and...
PUM
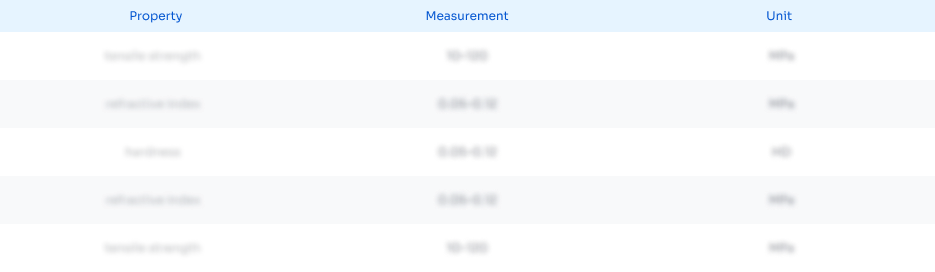
Abstract
Description
Claims
Application Information
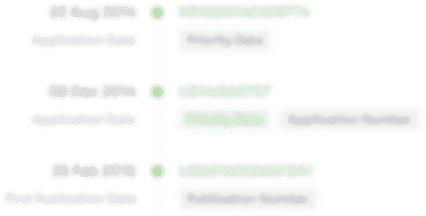
- R&D Engineer
- R&D Manager
- IP Professional
- Industry Leading Data Capabilities
- Powerful AI technology
- Patent DNA Extraction
Browse by: Latest US Patents, China's latest patents, Technical Efficacy Thesaurus, Application Domain, Technology Topic, Popular Technical Reports.
© 2024 PatSnap. All rights reserved.Legal|Privacy policy|Modern Slavery Act Transparency Statement|Sitemap|About US| Contact US: help@patsnap.com