Wind power prediction method based on hybrid cross-correlation entropy long short-term memory network
A technology of wind power forecasting and long-term short-term memory, which is applied in forecasting, neural learning methods, biological neural network models, etc., and can solve the problems of low accuracy and robustness of forecasting results
- Summary
- Abstract
- Description
- Claims
- Application Information
AI Technical Summary
Problems solved by technology
Method used
Image
Examples
Embodiment
[0122] Considering that the wind power sequence fluctuates strongly and is affected by seasonal factors. In the implementation case, the relevant data of four typical months (January, April, July, and October) in spring, summer, autumn and winter of a demonstration decentralized wind farm in a certain place were collected at a time interval of 5 minutes.
[0123] Step 1. Taking October data as an example, the original wind power sequence is adaptively decomposed into 16 subsequences;
[0124] Step 2, using the sample entropy function to reconstruct the 16 subsequences into 4 new feature sequences;
[0125] Step 3, normalize the wind speed, new characteristic power sequence, temperature and wind direction cosine;
[0126] Step 4. Construct PMC-LSTM prediction models for the four new feature sequences, and obtain four reconstructed sequence prediction values. The relevant parameters are given in Table 1;
[0127] Table 1 Data-related parameter settings for October
[0128] ...
PUM
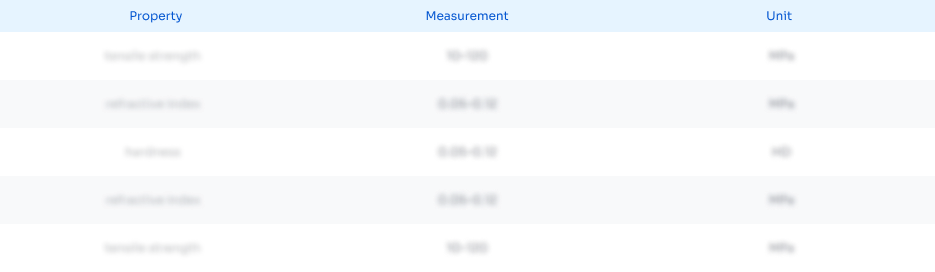
Abstract
Description
Claims
Application Information
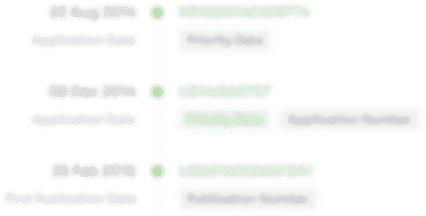
- Generate Ideas
- Intellectual Property
- Life Sciences
- Materials
- Tech Scout
- Unparalleled Data Quality
- Higher Quality Content
- 60% Fewer Hallucinations
Browse by: Latest US Patents, China's latest patents, Technical Efficacy Thesaurus, Application Domain, Technology Topic, Popular Technical Reports.
© 2025 PatSnap. All rights reserved.Legal|Privacy policy|Modern Slavery Act Transparency Statement|Sitemap|About US| Contact US: help@patsnap.com