No-reference video quality evaluation method based on deep learning
A video quality, deep learning technology, applied in the field of computer vision, can solve the problems of difficulty in training models, lack of versatility, insufficient quantity, etc., to solve technical difficulties and achieve the effect of evaluation
- Summary
- Abstract
- Description
- Claims
- Application Information
AI Technical Summary
Problems solved by technology
Method used
Image
Examples
Embodiment Construction
[0041] The present invention will be further described below in conjunction with accompanying drawing.
[0042] The method of the present invention includes a pre-trained convolutional neural network, a bidirectional GRU network and a video quality prediction network fused with time domain features. Assuming a video has T frames, the input to the model is parallel T frames of video frames. First, the pre-trained convolutional neural network extracts the content-aware features of each frame, processes the features with global pooling, discards redundant information, and preserves change information. Then, the fully connected layer is used to reduce the feature dimension, and the time domain features before and after are fused with the bidirectional GRU network. Finally, frame quality scores are computed using fully-connected layers, which are pooled over overall video quality to produce prediction scores. The network model provided by the method model fully and effectively co...
PUM
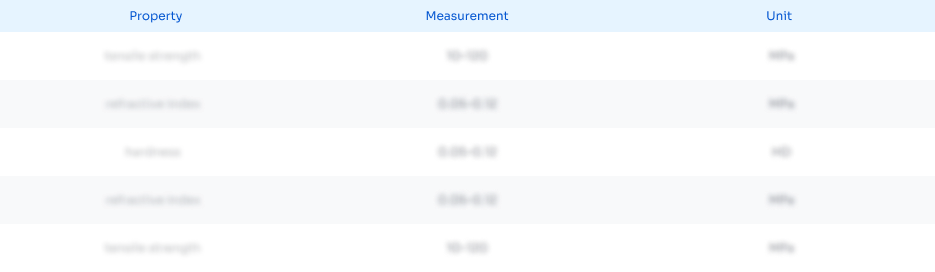
Abstract
Description
Claims
Application Information
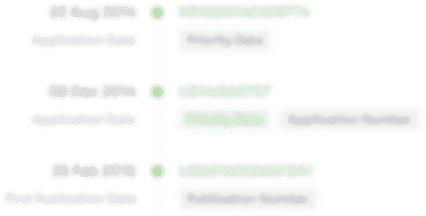
- R&D Engineer
- R&D Manager
- IP Professional
- Industry Leading Data Capabilities
- Powerful AI technology
- Patent DNA Extraction
Browse by: Latest US Patents, China's latest patents, Technical Efficacy Thesaurus, Application Domain, Technology Topic, Popular Technical Reports.
© 2024 PatSnap. All rights reserved.Legal|Privacy policy|Modern Slavery Act Transparency Statement|Sitemap|About US| Contact US: help@patsnap.com