Parameter self-learning interference suppression method based on expanded deep network
A deep network and interference suppression technology, applied in the field of signal processing, can solve problems such as overfitting, relatively high requirements for the quantity and quality of training data, and lack of interpretability, and achieve the effect of reducing complexity
- Summary
- Abstract
- Description
- Claims
- Application Information
AI Technical Summary
Problems solved by technology
Method used
Image
Examples
Embodiment Construction
[0034] The present invention will be further described below in conjunction with the accompanying drawings and embodiments.
[0035] The present invention will be described in further detail below in conjunction with the accompanying drawings and specific embodiments. The specific embodiment here is only one of the cases of the method in this paper, and it is impossible to include all the embodiments. Through the specific embodiments, the content of this part will make the staff in this field understand the working principle and specific steps of the method more clearly and implementation effects;
[0036] step 1: figure 1 Shown is the general flow chart of the parameter self-learning interference suppression method based on the expanded deep network according to an embodiment of the present invention. The original echo signal containing interference is sequentially subjected to short-time Fourier transform to obtain the time-spectrum data matrix Among them, 4096 and 256 a...
PUM
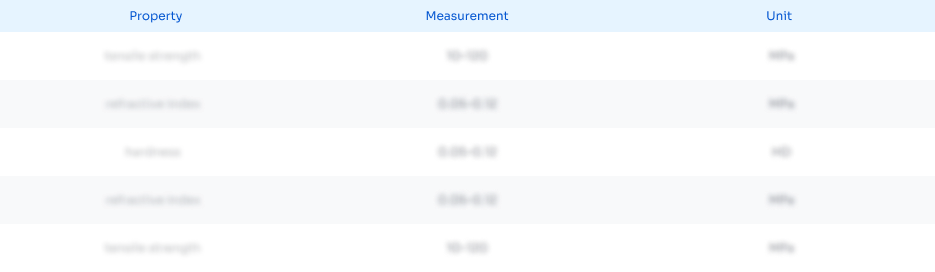
Abstract
Description
Claims
Application Information
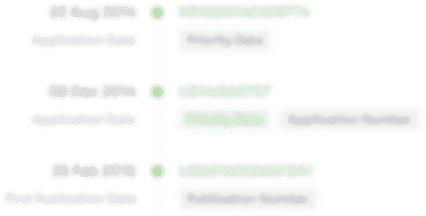
- R&D
- Intellectual Property
- Life Sciences
- Materials
- Tech Scout
- Unparalleled Data Quality
- Higher Quality Content
- 60% Fewer Hallucinations
Browse by: Latest US Patents, China's latest patents, Technical Efficacy Thesaurus, Application Domain, Technology Topic, Popular Technical Reports.
© 2025 PatSnap. All rights reserved.Legal|Privacy policy|Modern Slavery Act Transparency Statement|Sitemap|About US| Contact US: help@patsnap.com