Defect detection device and method based on distillation learning mechanism, and storage medium
A defect detection and learning mechanism technology, applied in the field of anomaly detection, can solve problems such as difficulty in collection, achieve the effects of simple deployment, optimized expression capabilities, and increased differences
- Summary
- Abstract
- Description
- Claims
- Application Information
AI Technical Summary
Problems solved by technology
Method used
Image
Examples
Embodiment 1
[0061] A defect detection device based on a distillation learning mechanism, including an acquisition module, a training module, and a detection module; the acquisition module is used to collect flawless industrial product image samples and form a training set; the training module is used to use the training set Train the network model, the network model includes a teacher model and a student model with the same backbone network structure, adopt the teacher model to guide the student model, and finally obtain an optimized network model; the detection module is used to input the image to be tested into the optimized network model and Output the detection result.
[0062] Such as figure 1 As shown, the complexity of the network layer of the teacher model is higher than the complexity of the network layer of the student model, and the middle layer between the teacher model and the student model is added with a feature information grafting module, which is used for synchronizing t...
Embodiment 2
[0066] This embodiment is optimized on the basis of embodiment 1, such as figure 2 As shown, the feature information grafting module includes a feature information difference value calculation part and a grafted feature information calculation part, and the feature information difference value calculation part is used to carry out the feature information of the residual combination blocks of the teacher model and the student model at the same level The difference value calculation, such as image 3 As shown, when the difference value is greater than the threshold value, enter the grafting feature information calculation part, fuse the teacher model feature information with the student model feature information, and then replace the original student model feature information, when the difference value is less than or equal to the threshold value , the feature information of the student model will not be replaced.
[0067] Further, as image 3 As shown, after the feature info...
Embodiment 3
[0084] This embodiment is optimized on the basis of embodiment 1 or 2, as figure 1 As shown, the backbone networks of the teacher model and the student model respectively include a convolution layer, a batch normalization layer, an activation function layer, and several residual modules arranged sequentially from front to back. The activation function layer uses parameters to modify the linear unit layer.
[0085]Further, the teacher model adopts the ResNet101 structure, the student model adopts the ResNet20 structure, the teacher model and the student model respectively include 4 residual combination blocks, and the number of residual modules contained in the 4 residual combination blocks of the teacher model The order is 6, 12, 24, 6, and the number of residual modules contained in the 4 residual combination blocks of the student model is 1, 2, 4, 1 in order.
[0086] Other parts of this embodiment are the same as those of Embodiment 1 or 2 above, so details are not repeate...
PUM
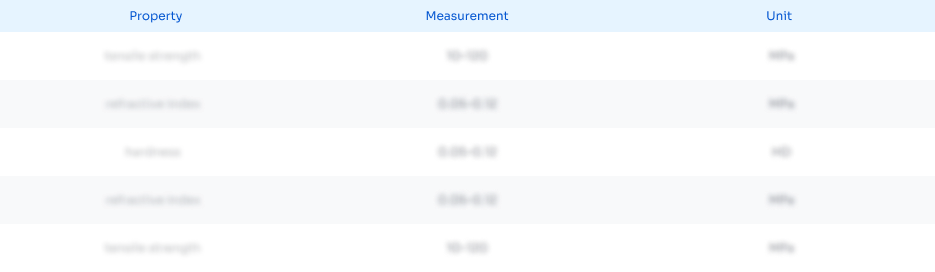
Abstract
Description
Claims
Application Information
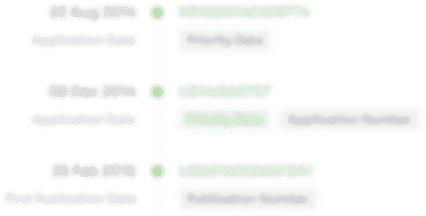
- R&D
- Intellectual Property
- Life Sciences
- Materials
- Tech Scout
- Unparalleled Data Quality
- Higher Quality Content
- 60% Fewer Hallucinations
Browse by: Latest US Patents, China's latest patents, Technical Efficacy Thesaurus, Application Domain, Technology Topic, Popular Technical Reports.
© 2025 PatSnap. All rights reserved.Legal|Privacy policy|Modern Slavery Act Transparency Statement|Sitemap|About US| Contact US: help@patsnap.com