Electrocardiosignal feature point detection method and system
A feature point detection and electrocardiographic signal technology, applied in the field of artificial intelligence data analysis, can solve the problems of difficult detection of P waves and T waves, and changeable shapes of P waves and T waves, so as to improve the robustness and accuracy. Effect
- Summary
- Abstract
- Description
- Claims
- Application Information
AI Technical Summary
Problems solved by technology
Method used
Image
Examples
Embodiment 1
[0040] Such as figure 1 As shown, a normal cardiac cycle is usually composed of P wave, QRS wave group and T wave. The analysis of the ECG is to find the QRS wave group first, because the QRS wave group is the most prominent feature in the ECG and is the basis for the detection of other waveforms. . According to the defects of the existing monitoring method described in the background technology, this embodiment proposes an automatic detection method of ECG signal feature points based on deep learning technology;
[0041] Deep learning is gradually being used in the detection of ECG signal feature points. Deep learning has strong self-learning ability and highly nonlinear mapping characteristics, and can learn ECG signal features from a large amount of training data. Currently, based on traditional machine learning The ECG feature point detection algorithm of the technology requires pre-specified thresholds or other assumptions. This embodiment proposes an automatic detection...
Embodiment 2
[0130] This embodiment provides a system for detecting ECG feature points, including:
[0131] A signal acquisition module configured to acquire ECG signals in continuous time;
[0132] The quality evaluation module is configured to evaluate the quality of the ECG signals in continuous time, and judge whether denoising processing is required;
[0133] The denoising module is configured to perform denoising processing on ECG signals in continuous time to correct baseline drift;
[0134] The segmentation processing module is configured to segment and process the ECG signals in continuous time to obtain standard ECG signal segments;
PUM
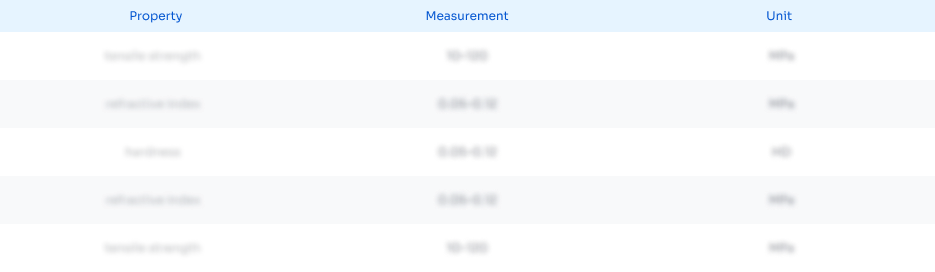
Abstract
Description
Claims
Application Information
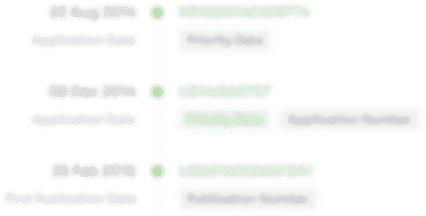
- R&D Engineer
- R&D Manager
- IP Professional
- Industry Leading Data Capabilities
- Powerful AI technology
- Patent DNA Extraction
Browse by: Latest US Patents, China's latest patents, Technical Efficacy Thesaurus, Application Domain, Technology Topic.
© 2024 PatSnap. All rights reserved.Legal|Privacy policy|Modern Slavery Act Transparency Statement|Sitemap