Ultra-short-term wind power prediction method based on DCCSO optimization deep learning model
A technology for wind power forecasting and deep learning, applied in neural learning methods, genetic models, forecasting, etc., can solve problems such as long running time, improve time sensitivity, improve convergence speed and generalization performance, and improve wind power forecasting. The effect of precision
- Summary
- Abstract
- Description
- Claims
- Application Information
AI Technical Summary
Problems solved by technology
Method used
Image
Examples
Embodiment 1
[0022] Such as figure 1 Shown is an embodiment of the ultra-short-term wind power prediction method based on the DCCSO optimization deep learning model of the present invention, the method includes the following steps:
[0023] S10. Collect the historical data of original wind power, original wind speed and original wind direction, and preprocess the original wind power, original wind speed and original wind direction historical data to obtain wind power time series, wind speed time series and wind direction time series;
[0024] S20. Converting the wind direction time series in step S10 to a sine sequence of wind direction and a cosine sequence of wind direction;
[0025] S30. Splicing the wind power time series in step S10, the wind speed time series, the sine sequence of wind direction and the cosine sequence of wind direction in step S20 and forming a single input sample sequence X=[x with a time step size of T 1 ,x 2 ,...,x n ], where x k (1≤k≤n) is a time-dimension v...
Embodiment 2
[0100] This embodiment is an embodiment of a specific application of Embodiment 1. In this embodiment:
[0101] In step S10, the historical data of original wind power, original wind speed and original wind direction is the wind power, wind speed and wind direction data collected continuously for one month and collected once every 10 minutes, and there are 144 data points (including wind power, wind speed and wind direction) every day .
[0102] In step S40, the training samples are the first 3320 historical wind power data.
[0103] In step S70, the temporal attention-gated recurrent unit deep learning prediction model trained in step S60 is used to predict wind power power 10 minutes in advance.
[0104] Such as figure 2 As shown, in this embodiment, the CSO-optimized TA-GRU prediction model is used as a comparison, and the prediction result of the CSO-optimized TA-GRU prediction model, the prediction result of the DCCSO-optimized TA-GRU prediction model, and the actual v...
PUM
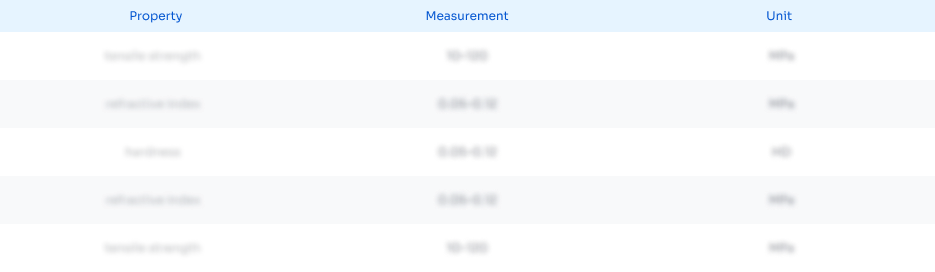
Abstract
Description
Claims
Application Information
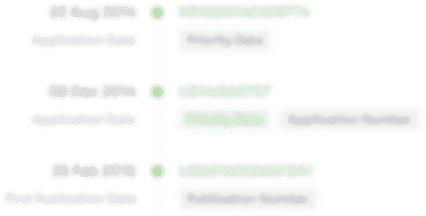
- R&D Engineer
- R&D Manager
- IP Professional
- Industry Leading Data Capabilities
- Powerful AI technology
- Patent DNA Extraction
Browse by: Latest US Patents, China's latest patents, Technical Efficacy Thesaurus, Application Domain, Technology Topic, Popular Technical Reports.
© 2024 PatSnap. All rights reserved.Legal|Privacy policy|Modern Slavery Act Transparency Statement|Sitemap|About US| Contact US: help@patsnap.com