Tea disease identification method based on deep transfer learning
A transfer learning and disease identification technology, applied in the field of deep learning, can solve the problems of not being able to learn the correlation coefficient adaptively, and not considering the correlation of different feature channels, etc., so as to improve the recognition accuracy, high model recognition speed, and accurate recognition high rate effect
- Summary
- Abstract
- Description
- Claims
- Application Information
AI Technical Summary
Problems solved by technology
Method used
Image
Examples
Embodiment Construction
[0050] In order to make the object, technical solution and advantages of the present invention clearer, the present invention will be further described in detail below in conjunction with the accompanying drawings and embodiments. It should be understood that the specific embodiments described here are only used to explain the present invention, not to limit the present invention.
[0051] The tea disease identification method based on depth transfer learning of the embodiment of the present invention, the method comprises the following steps:
[0052] Training phase:
[0053] Step 1: Obtain pictures of multiple varieties of tea leaves and corresponding diseases as a training data set;
[0054] The source area of the experimental data of the present invention is a certain tea garden base, which has planted more than 10 varieties of tea. The tea diseases in the base are tea white spot disease, tea ring spot disease, tea coal disease, tea round red spot disease and tea leaf ...
PUM
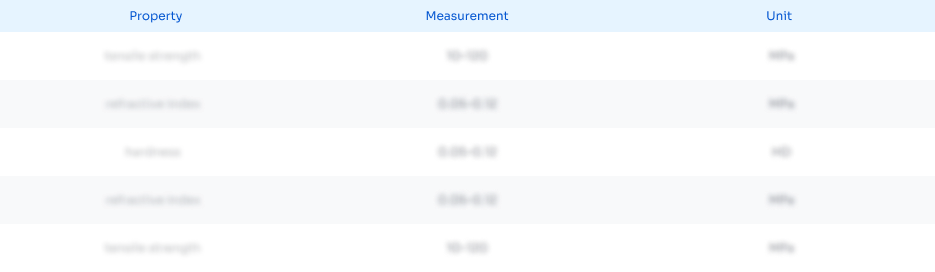
Abstract
Description
Claims
Application Information
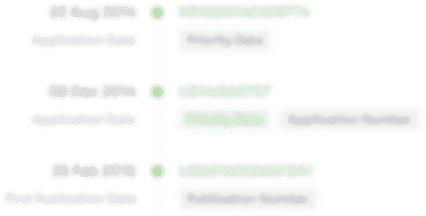
- R&D Engineer
- R&D Manager
- IP Professional
- Industry Leading Data Capabilities
- Powerful AI technology
- Patent DNA Extraction
Browse by: Latest US Patents, China's latest patents, Technical Efficacy Thesaurus, Application Domain, Technology Topic, Popular Technical Reports.
© 2024 PatSnap. All rights reserved.Legal|Privacy policy|Modern Slavery Act Transparency Statement|Sitemap|About US| Contact US: help@patsnap.com