Improved semi-supervised image classification method, equipment and storage medium
A classification method and semi-supervised technology, applied in neural learning methods, instruments, biological neural network models, etc., can solve the problems of uncontrollable models, difficult convergence, model collapse, etc., to improve the discrimination ability, low error rate, and good testing. The effect of error rate
- Summary
- Abstract
- Description
- Claims
- Application Information
AI Technical Summary
Problems solved by technology
Method used
Image
Examples
Embodiment 1
[0079] An improved semi-supervised image classification method based on Triple-Gan, comprising the following steps:
[0080] (1) Build an improved semi-supervised image classification model; as figure 1 As shown, the improved semi-supervised image classification model includes a generator, a discriminator and a set of sub-judgers, and a set of sub-judgers includes the first sub-judger D s1 and the second sub-determinator D s2 ;
[0081] The generator G draws from the distribution P z Extract random noise z as input, and train simultaneously with all judgers in a way of generating confrontation; judger D 0 is the discriminative part of the improved semi-supervised image classification model, which divides the input image into the correct category as much as possible; a set of sub-judgers includes the first sub-judger D s1 and the second sub-determinator D s2 , except for the last few layers, they and the discriminator D 0 There are roughly the same network layers and para...
Embodiment 2
[0149] A computer device includes a memory and a processor, the memory stores a computer program, and the processor implements the steps of the Triple-Gan-based improved semi-supervised image classification method described in Embodiment 1 when executing the computer program.
Embodiment 3
[0151] A computer-readable storage medium, on which a computer program is stored. When the computer program is executed by a processor, the steps of the Triple-Gan-based improved semi-supervised image classification method described in Embodiment 1 are implemented.
PUM
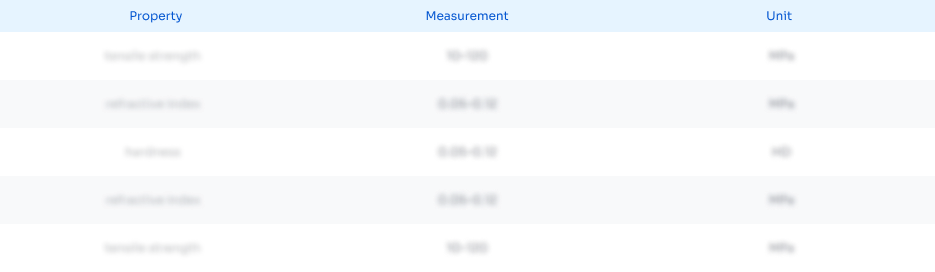
Abstract
Description
Claims
Application Information
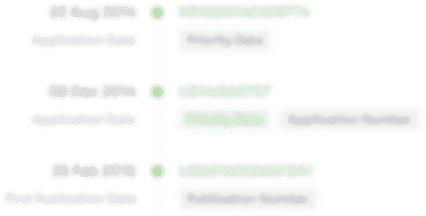
- R&D Engineer
- R&D Manager
- IP Professional
- Industry Leading Data Capabilities
- Powerful AI technology
- Patent DNA Extraction
Browse by: Latest US Patents, China's latest patents, Technical Efficacy Thesaurus, Application Domain, Technology Topic.
© 2024 PatSnap. All rights reserved.Legal|Privacy policy|Modern Slavery Act Transparency Statement|Sitemap