Digestive endoscopy simulator inspection quality evaluation method and system
A technology of digestive endoscopy and simulators, which is applied to instruments, character and pattern recognition, biological neural network models, etc., to improve the efficiency and accuracy of evaluation and improve the operation level
- Summary
- Abstract
- Description
- Claims
- Application Information
AI Technical Summary
Problems solved by technology
Method used
Image
Examples
Embodiment 1
[0048] This embodiment 1 provides an evaluation system for the operation level of a digestive endoscopy simulator, which includes:
[0049] The recognition module is used to use the pre-trained classification recognition model to process the images acquired during the digestive endoscopy process and identify the inspection site;
[0050] The first calculation module is used to calculate the evaluation index according to the identified inspection site; wherein, the evaluation index includes inspection coverage, detection rate of key parts, inspection time, operation technique score and case diagnosis accuracy;
[0051] The second calculation module is used to perform fusion weighted calculation on the inspection coverage rate, detection rate of key parts, inspection time, operation technique score and case diagnosis accuracy rate to obtain the final operation score;
[0052] The judging module is used for judging the operation level according to the final operation score. The ...
Embodiment 2
[0072] This embodiment 2 provides a method for evaluating the operation level of a digestive endoscope simulator, the method comprising:
[0073] Use the pre-trained classification recognition model to process the images acquired during digestive endoscopy to identify the inspection site;
[0074] Calculate the evaluation index according to the identified inspection site; wherein, the evaluation index includes inspection coverage, detection rate of key parts, inspection time, operation technique score, and case diagnosis accuracy;
[0075] Fusion and weighted calculation is performed on the inspection coverage rate, detection rate of key parts, inspection time, operation technique score and case diagnosis accuracy to obtain the final operation score;
[0076] According to the final operation score, determine the level of operation.
[0077] In Embodiment 2, the pre-trained classification and recognition model is trained from a training set; the training set includes a plurali...
Embodiment 3
[0093] In this embodiment 3, a deep learning-based intelligent evaluation method for the operation and inspection quality of a digestive endoscope simulator is provided. From the coverage of gastroscopy (comprehensive evaluation), the detection rate of key parts (accuracy evaluation), the time of gastroscopy (rapid evaluation), the operation method of gastroscopy (standard evaluation), and pathological diagnosis, multimodal fusion algorithm learning and Predict and evaluate the user's operation level; in addition, record the user's historical operation level, draw the historical learning curve, and evaluate the user's operation learning level.
[0094] The gastroscopy coverage rate is to classify the representative pictures of various parts of the stomach taken by the user through the DCNN picture classification model, and calculate the number of observed parts and the loss rate of each part.
[0095] The coverage rate of the gastroscopy is specifically defined as: the ratio o...
PUM
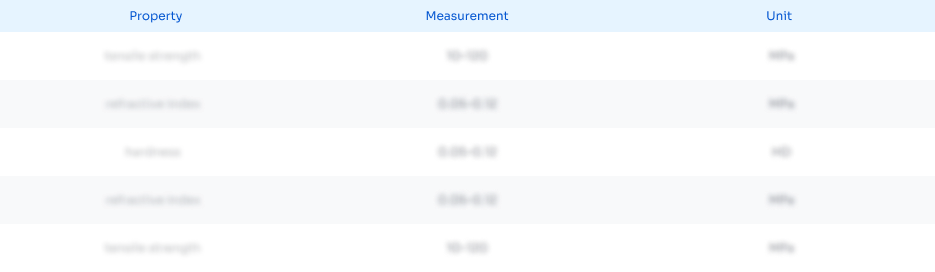
Abstract
Description
Claims
Application Information
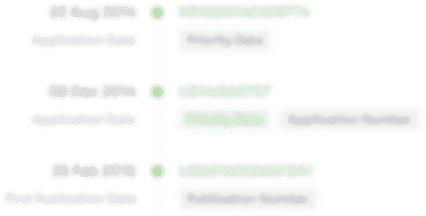
- R&D Engineer
- R&D Manager
- IP Professional
- Industry Leading Data Capabilities
- Powerful AI technology
- Patent DNA Extraction
Browse by: Latest US Patents, China's latest patents, Technical Efficacy Thesaurus, Application Domain, Technology Topic.
© 2024 PatSnap. All rights reserved.Legal|Privacy policy|Modern Slavery Act Transparency Statement|Sitemap