Traffic sign recognition model training method, traffic sign recognition method and device
A traffic sign recognition and model training technology, applied in the field of artificial intelligence, can solve problems such as increasing the computational complexity of the model, and achieve the effects of fast recognition, reduced loss, and improved accuracy and real-time performance.
- Summary
- Abstract
- Description
- Claims
- Application Information
AI Technical Summary
Problems solved by technology
Method used
Image
Examples
Embodiment 1
[0034] Improved real-time traffic sign recognition model training method based on FCOS algorithm, such as figure 1 As shown, the overall model network structure is as follows figure 2 As shown, it specifically includes the following steps:
[0035] Step 1: Initialize and set the positive sample threshold to 0.3, the training epoch to 100, and the batch size to 8, and use ImageNet pre-training weights for initialization, and set the number of pixels on the short side of the input image to 800, and the number of pixels on the long side Less than or equal to 1333.
[0036] Step 2: ResNet-50 is used as the backbone network to extract features from the pictures in the TT100K dataset (Tsinghua Traffic Sign Database), and the CABM module is added to the first and last layers of the ResNet-50 network. After the above operations, The final C3, C4, C5 feature map;
[0037] In the improved ResNet-50 network, the swish activation function is used to replace the ReLu activation functio...
Embodiment 2
[0057] A traffic sign recognition method, which puts the picture to be recognized into the above-mentioned trained model for prediction to obtain the final recognition effect.
[0058] In order to verify the prediction effect of the method proposed in the present invention, four different road scene pictures are randomly selected for recognition, and the recognition effect diagram is as follows image 3 As shown, and the recognition time of each picture is 41ms.
[0059] The model of the present invention adds the CBAM module and adopts the swish activation function in the ResNet-50 network. Experiments show that, under the situation of not affecting the recognition speed, adopting the model of the present invention to carry out traffic sign recognition is comparable to the existing conventional ResNet-50 network. Models are compared. Here, the conventional ResNet-50 network model is used as a comparison example. Specifically, the literature He K, Zhang X, Ren S, et al.Deep Re...
Embodiment 3
[0063] The present invention further provides a traffic sign recognition device, which includes a memory, a processor, and a computer program stored in the memory and operable on the processor. When the processor executes the program, the steps of the above method are realized.
PUM
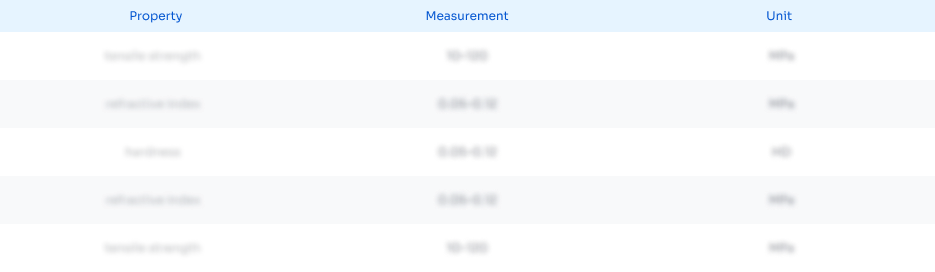
Abstract
Description
Claims
Application Information
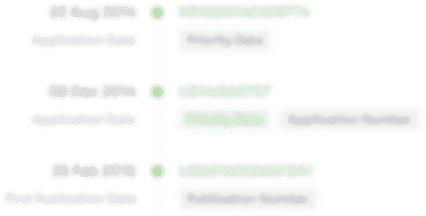
- R&D Engineer
- R&D Manager
- IP Professional
- Industry Leading Data Capabilities
- Powerful AI technology
- Patent DNA Extraction
Browse by: Latest US Patents, China's latest patents, Technical Efficacy Thesaurus, Application Domain, Technology Topic, Popular Technical Reports.
© 2024 PatSnap. All rights reserved.Legal|Privacy policy|Modern Slavery Act Transparency Statement|Sitemap|About US| Contact US: help@patsnap.com