Park microgrid load optimization scheduling method and system based on two-stage reinforcement learning
A technology of reinforcement learning and scheduling methods, applied in machine learning, information technology support systems, instruments, etc., can solve problems such as efficient load optimization scheduling, incomplete acquisition of user information and environmental information, etc., to save energy consumption and improve availability. Scalability, the effect of saving production costs
- Summary
- Abstract
- Description
- Claims
- Application Information
AI Technical Summary
Problems solved by technology
Method used
Image
Examples
Embodiment 1
[0079] In the first aspect, the present invention first proposes a two-stage reinforcement learning-based load optimization scheduling method for park microgrids, see figure 1 , the method includes:
[0080] S1. Obtain relevant parameters of each microgrid in the park; the relevant parameters include environmental parameters, load demand data, and electricity price data in the electricity wholesale market;
[0081] S2. The load agent obtains the optimal price of each microgrid by using the reinforcement learning algorithm based on the stochastic policy gradient based on the relevant parameters;
[0082] S3. Based on the optimal price, use the deep reinforcement learning Actor-Critic algorithm to optimize the scheduling of each microgrid in the park.
[0083] It can be seen that this embodiment obtains the relevant parameters of each microgrid in the park, and then the load agent uses the reinforcement learning algorithm based on stochastic policy gradient to obtain the optima...
Embodiment 2
[0136] In the second aspect, the present invention also provides a park microgrid load optimization scheduling system based on two-stage reinforcement learning, see figure 2 , the system consists of:
[0137] The relevant parameter acquisition module is used to obtain the relevant parameters of each micro-grid in the park; the relevant parameters include environmental parameters, load demand data, and electricity price data in the electricity wholesale market;
[0138] The load agent optimization decision module is used for the load agent to obtain the optimal price of each microgrid based on the relevant parameters using the reinforcement learning algorithm based on the stochastic policy gradient;
[0139] The park micro-grid optimization scheduling module is used to optimize the scheduling of each micro-grid in the park by using the deep reinforcement learning Actor-Critic algorithm based on the optimal price.
[0140] Optionally, the system further includes: a data prepro...
PUM
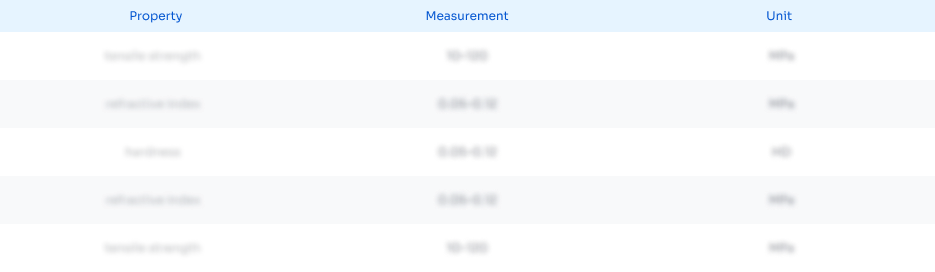
Abstract
Description
Claims
Application Information
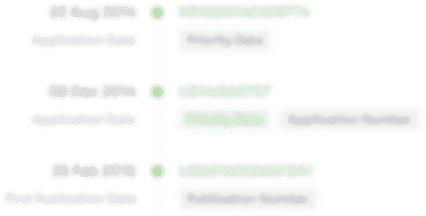
- R&D Engineer
- R&D Manager
- IP Professional
- Industry Leading Data Capabilities
- Powerful AI technology
- Patent DNA Extraction
Browse by: Latest US Patents, China's latest patents, Technical Efficacy Thesaurus, Application Domain, Technology Topic, Popular Technical Reports.
© 2024 PatSnap. All rights reserved.Legal|Privacy policy|Modern Slavery Act Transparency Statement|Sitemap|About US| Contact US: help@patsnap.com