A Denoising Method Based on Multi-Branch Selective Kernel Nested Connection Residual Networks
A nested connection and selective technology, applied in neural learning methods, biological neural network models, instruments, etc., can solve problems such as noise suppression of complex seismic data that cannot be adaptively processed, and achieve high computing efficiency and denoising performance. The effect of reducing residual connections and improving computational efficiency
- Summary
- Abstract
- Description
- Claims
- Application Information
AI Technical Summary
Problems solved by technology
Method used
Image
Examples
Embodiment Construction
[0036] In order to enable those skilled in the art to better understand the technical solutions of the present invention, the present invention will be further described in detail below in conjunction with the accompanying drawings and specific embodiments.
[0037] Such as Figure 1-5 As shown, a denoising method based on a multi-branch selective kernel nested connection residual network. It should be noted that the residual network is a network architecture proposed for the difficulty of training deep convolutional neural networks. The problem of network degradation caused by the increase of depth, but the number of network layers is large, and the calculation efficiency needs to be improved; the residual network consists of a series such as figure 2 The Residual Module (Residual Module, ResM) in b is composed, which is in figure 2 Add identity mapping (Identity Mapping, IM) on the basis of the Convolution Neural Network Module (CNNM) in a, that is, f(x)=x; when the netwo...
PUM
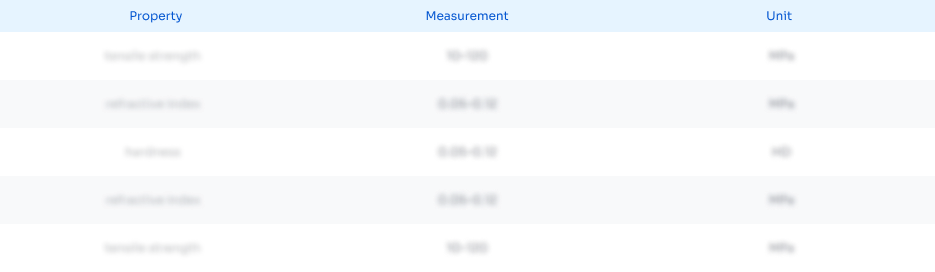
Abstract
Description
Claims
Application Information
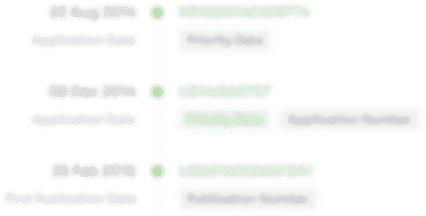
- R&D
- Intellectual Property
- Life Sciences
- Materials
- Tech Scout
- Unparalleled Data Quality
- Higher Quality Content
- 60% Fewer Hallucinations
Browse by: Latest US Patents, China's latest patents, Technical Efficacy Thesaurus, Application Domain, Technology Topic, Popular Technical Reports.
© 2025 PatSnap. All rights reserved.Legal|Privacy policy|Modern Slavery Act Transparency Statement|Sitemap|About US| Contact US: help@patsnap.com