Hyperspectral classification method combining graph structure and convolutional neural network
A technology of convolutional neural network and hyperspectral classification, which is applied in the field of hyperspectral image classification to achieve the effects of improving classification accuracy, improving adaptability and suppressing noise
- Summary
- Abstract
- Description
- Claims
- Application Information
AI Technical Summary
Problems solved by technology
Method used
Image
Examples
Embodiment Construction
[0053] Now in conjunction with accompanying drawing, specific embodiment of the present invention is described in further detail as follows:
[0054] The experiment of the present invention uses GeForce GTX 1080Ti 11G GPU and 3.70G Intel i9-10900K CPU on the computer, and trains the present invention in pytorch 1.8. The invention is used for classification on Pavia University, Salinas and Houston 2013 datasets.
[0055] First of all, the original hyperspectral image is segmented by superpixels to extract the spectral features of each superpixel block; then a multi-scale graph with superpixels as nodes is constructed; then the graph network is used to extract features from the multi-scale graph, The product neural network is used to extract the spectral features; finally, the features are fused, and the image features are interpreted by using the cross-entropy loss to obtain the label of each pixel and predict the node.
[0056] The method of the present invention mainly inclu...
PUM
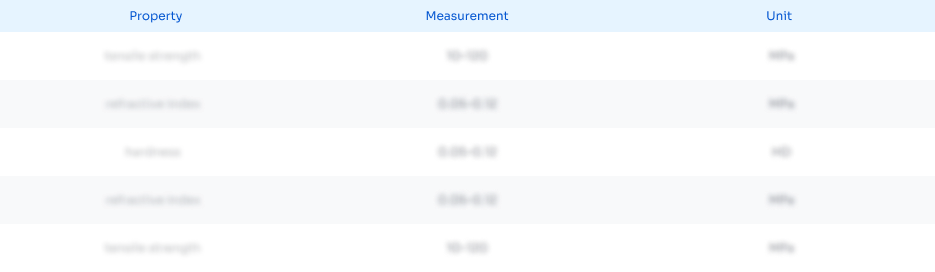
Abstract
Description
Claims
Application Information
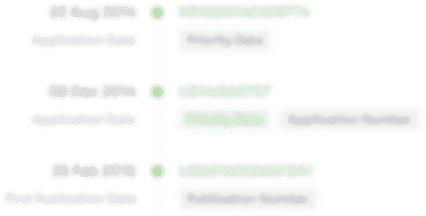
- R&D Engineer
- R&D Manager
- IP Professional
- Industry Leading Data Capabilities
- Powerful AI technology
- Patent DNA Extraction
Browse by: Latest US Patents, China's latest patents, Technical Efficacy Thesaurus, Application Domain, Technology Topic, Popular Technical Reports.
© 2024 PatSnap. All rights reserved.Legal|Privacy policy|Modern Slavery Act Transparency Statement|Sitemap|About US| Contact US: help@patsnap.com