WOA-QRLSTM reservoir inflow probabilistic prediction method based on mean shift clustering
A technology of inflow and mean shift, applied in forecasting, neural learning methods, biological neural network models, etc., can solve the problems of difficulty in obtaining reservoir inflow forecast results, low forecast accuracy, and unsatisfactory forecast effects. Quantify uncertainty, improve accuracy and stability, improve the effect of accuracy
- Summary
- Abstract
- Description
- Claims
- Application Information
AI Technical Summary
Problems solved by technology
Method used
Image
Examples
Embodiment Construction
[0026] In this embodiment, a method based on mean drift clustering and WOA-QRLSTM predictive model is predictive, such as figure 1 As shown, it is performed as follows:
[0027] Step 1. Collect the reservoir warehousing traffic data and affect the factor data of the reservoir's storage traffic and preprocess, resulting in the processing data set DataSet = {[w (t), g m (t)] | T = 1, 2, ..., t; m = 1, 2, ..., m}, where W (t) represents the reservoir flow rate of the T date; M represents the reservoir Number of factors of the library flow, g m (t) Indicates the value of the mth impact factor in the dayt day; T represents the total number of collected days;
[0028] Step 2, at a time interval at a certain time period D, divide the data set after the pre-treated data, to obtain I group sample data {dataGRuop i | i = 1, 2, ..., i}, and I meet [T / D], where DataGroup i Indicates Group I sample data, and DataGroup i = [W '(i), g' m (i)], g ' m (i) = (g m (D × (i-1) +1), g m (D × (i-1) +2...
PUM
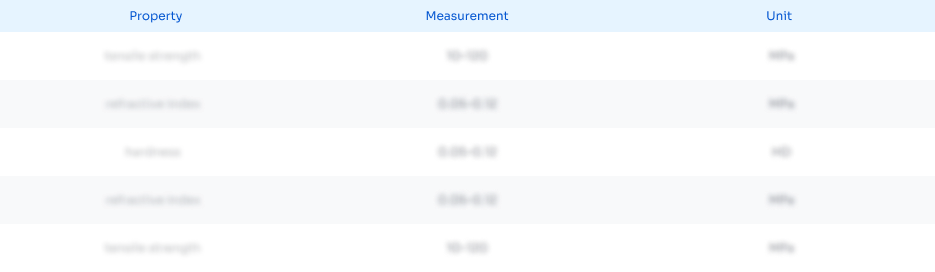
Abstract
Description
Claims
Application Information
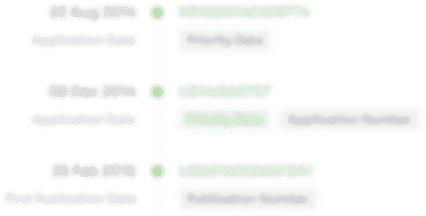
- R&D Engineer
- R&D Manager
- IP Professional
- Industry Leading Data Capabilities
- Powerful AI technology
- Patent DNA Extraction
Browse by: Latest US Patents, China's latest patents, Technical Efficacy Thesaurus, Application Domain, Technology Topic, Popular Technical Reports.
© 2024 PatSnap. All rights reserved.Legal|Privacy policy|Modern Slavery Act Transparency Statement|Sitemap|About US| Contact US: help@patsnap.com