Mixed time period mode multivariable time sequence prediction method based on neural network
A hybrid time and neural network technology, applied in the field of data forecasting based on deep learning, can solve the problem of not being able to solve multivariate forecasting tasks well, and achieve the effect of improving information utilization, promoting fusion, and alleviating forecast lag
- Summary
- Abstract
- Description
- Claims
- Application Information
AI Technical Summary
Problems solved by technology
Method used
Image
Examples
specific Embodiment approach 1
[0046] Specific implementation mode 1. Combination figure 1 and figure 2 As shown, the present invention provides a neural network-based mixed time-periodic mode multivariate time-series forecasting method, comprising,
[0047] The encoder is used to extract the features of the original input data of the mixed cycle, and after the feature extraction of multiple convolutional long-term short-term memory network units, the encoding fully connected layer and the vector merging unit included in the encoder, the length of the short cycle length + 3 is obtained. Future time series data characteristics;
[0048] Then the decoder processes the original input data of the mixed period and the characteristics of future time series data, and processes the data through multiple bidirectional long and short-term memory network units of the decoder, fusion attention mechanism, decoding fully connected layer, autoregressive model and comprehensive prediction unit After that, the final time...
specific Embodiment
[0164] The datasets that are usually faced are multivariate (multi-user) datasets, so it is necessary to consider that the scale of different variables may affect the quality of the evaluation. Use the following metrics to avoid this problem:
[0165] Empirical Correlation Coefficient (CORR):
[0166] ΔY it =Y it -mean(Y i ),
[0167]
[0168]
[0169] Root mean squared relative error (Root Relative Squared Error, RRSE):
[0170]
[0171] Relative Absolute Error (RAE):
[0172]
[0173] Ω Test represents the divided test set. RRSE and RAE are normalized versions of Root Mean Square Error (RMSE) and Mean Absolute Error (MAE), respectively, which avoid the interference caused by scale size in multivariate evaluations. For RRSE and RAE, lower values represent better predictions; for CORR, the opposite is true.
[0174] The forecast details and strategies are shown as follows:
[0175] According to the ratio of 0.6:0.2:0.2, the original data set is divided ...
PUM
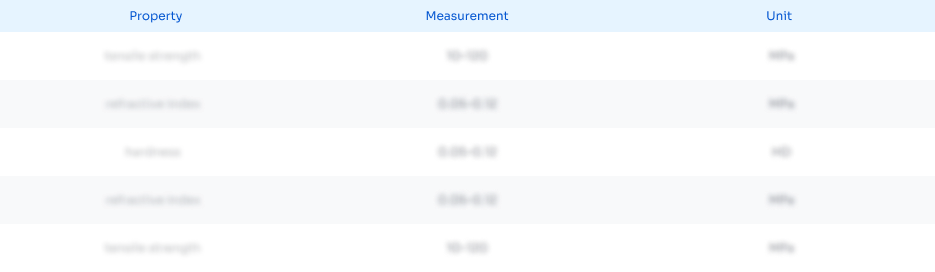
Abstract
Description
Claims
Application Information
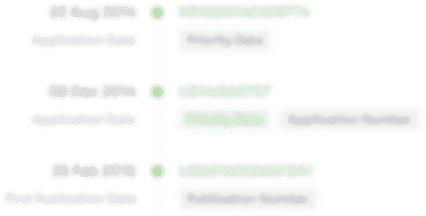
- Generate Ideas
- Intellectual Property
- Life Sciences
- Materials
- Tech Scout
- Unparalleled Data Quality
- Higher Quality Content
- 60% Fewer Hallucinations
Browse by: Latest US Patents, China's latest patents, Technical Efficacy Thesaurus, Application Domain, Technology Topic, Popular Technical Reports.
© 2025 PatSnap. All rights reserved.Legal|Privacy policy|Modern Slavery Act Transparency Statement|Sitemap|About US| Contact US: help@patsnap.com