Computer-aided diagnosis method for colorectal cancer based on small sample learning
A computer-aided, colorectal cancer technology, applied in the field of image processing, can solve the problems of limited deep learning application, high manual labeling cost, manual operation, etc., to improve generalization ability, improve accuracy and generalization, reduce high cost cost effect
- Summary
- Abstract
- Description
- Claims
- Application Information
AI Technical Summary
Problems solved by technology
Method used
Image
Examples
Embodiment Construction
[0017] The present invention will be described in further detail below in conjunction with the accompanying drawings and specific embodiments. It should be understood that the specific embodiments described here are only used to explain the present invention, not to limit the present invention.
[0018] Since deep learning-based models usually require massive data samples for learning, but large-scale data and data labeling require high labor and time costs, the development of deep learning based on small samples is particularly important for medical image processing. In addition, most of the current diagnostic methods for colorectal cancer rely on the personal experience of microscopes and pathologists, which makes the diagnostic results subjective and variable, and the existing algorithms for medical image segmentation are usually less robust. And the task is single, for example, usually only focus on segmentation, while ignoring the diagnosis of benign and malignant and dif...
PUM
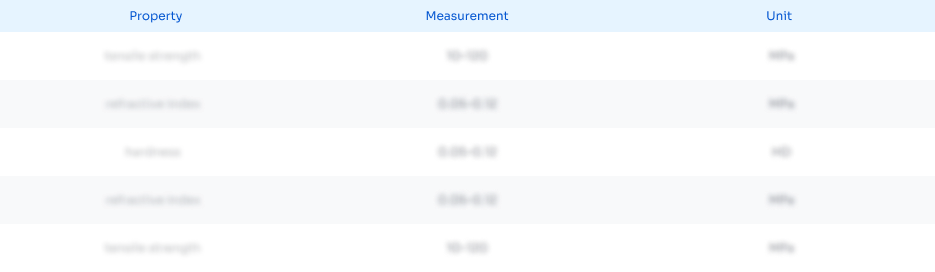
Abstract
Description
Claims
Application Information
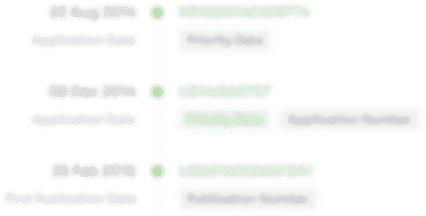
- R&D Engineer
- R&D Manager
- IP Professional
- Industry Leading Data Capabilities
- Powerful AI technology
- Patent DNA Extraction
Browse by: Latest US Patents, China's latest patents, Technical Efficacy Thesaurus, Application Domain, Technology Topic, Popular Technical Reports.
© 2024 PatSnap. All rights reserved.Legal|Privacy policy|Modern Slavery Act Transparency Statement|Sitemap|About US| Contact US: help@patsnap.com