Trajectory representation method and system based on deep learning
A technology of deep learning and trajectory, which is applied in neural learning methods, image data processing, 3D modeling, etc., can solve the problems of unreserved space-time attributes of guiding lines, and the inability of guiding lines to adapt to actual road scenes, so as to improve adaptability and automation degree of effect
- Summary
- Abstract
- Description
- Claims
- Application Information
AI Technical Summary
Problems solved by technology
Method used
Image
Examples
Embodiment 2
[0052] refer to Figure 7 , the second aspect of the present invention provides a trajectory representation system 1 based on deep learning, including: an acquisition module 11 for acquiring trajectory sampling point data, and using a neural network model to encode the sampling points in the trajectory into fixed-dimensional Vector; extraction module 12, for the trajectory sampling point after encoding, utilizes the convolution of described neural network model and pooling operation to extract spatial feature vector; Output module 13, is used for inputting described spatial feature vector to LSTM model In, the LSTM model obtains the trajectory semantic vector with spatio-temporal features by extracting the temporal features in the spatial feature vector.
[0053] Further, a reconstruction module is further included, the reconstruction module is used to decode and reconstruct the trajectory semantic vector of the spatio-temporal feature by using the trained decoder, and output ...
Embodiment 3
[0056] refer to Figure 8 , the third aspect of the present invention provides an electronic device, including: one or more processors; storage means for storing one or more programs, when the one or more programs are used by the one or more executed by one or more processors, such that the one or more processors implement the method of the first aspect of the present invention.
[0057] The electronic device 500 may include a processing device (such as a central processing unit, a graphics processing unit, etc.) 501, which may be loaded into a random access memory (RAM) 503 according to a program stored in a read-only memory (ROM) 502 or loaded from a storage device 508 Various appropriate actions and processing are performed by the program. In the RAM 503, various programs and data necessary for the operation of the electronic device 500 are also stored. The processing device 501 , ROM 502 and RAM 503 are connected to each other through a bus 504 . An input / output (I / O) i...
PUM
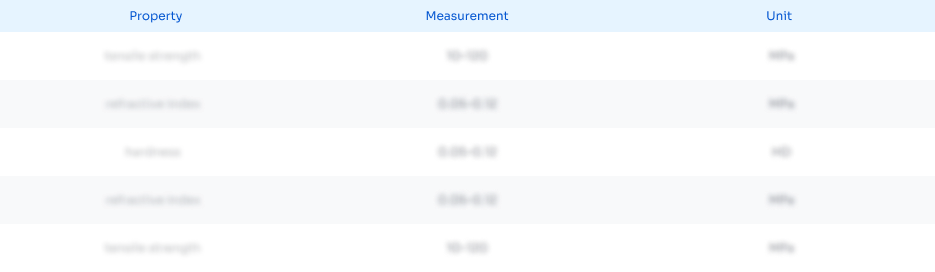
Abstract
Description
Claims
Application Information
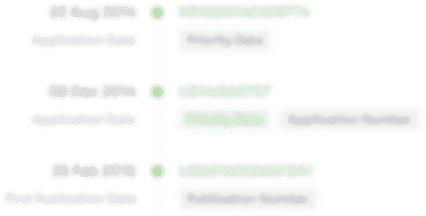
- R&D Engineer
- R&D Manager
- IP Professional
- Industry Leading Data Capabilities
- Powerful AI technology
- Patent DNA Extraction
Browse by: Latest US Patents, China's latest patents, Technical Efficacy Thesaurus, Application Domain, Technology Topic, Popular Technical Reports.
© 2024 PatSnap. All rights reserved.Legal|Privacy policy|Modern Slavery Act Transparency Statement|Sitemap|About US| Contact US: help@patsnap.com