User position prediction framework based on clustered graph federal learning
A user and federation technology, applied in the field of smart device user location prediction, can solve the problems of limited activity data, limited data volume, expensive and other problems, and achieve the effect of solving heterogeneity and solving the problem of insufficient training cost.
- Summary
- Abstract
- Description
- Claims
- Application Information
AI Technical Summary
Problems solved by technology
Method used
Image
Examples
Embodiment Construction
[0036] In order to make the purpose, technical solution and advantages of the present invention more clear, the present invention will be further described in detail below in conjunction with the accompanying drawings and examples. It should be understood that the specific embodiments described here are only used to explain the present invention, not to limit the present invention. This embodiment provides a user location prediction method based on clustering graph federated learning, the flow chart is as follows Figure 1-Figure 4 As shown, it mainly includes the following steps:
[0037] In step S1, the user uses the sequence prediction model locally for training, such as figure 2 shown. In this step, specifically include:
[0038] S11. First initialize the parameters of the model User's Embedded Representation iteration counter r a = 1, specify the number of iterations T of the user's local training a .
[0039] S12. Determine whether the current number of rounds r...
PUM
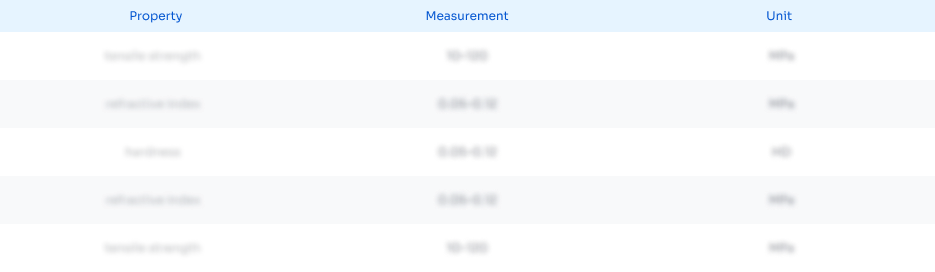
Abstract
Description
Claims
Application Information
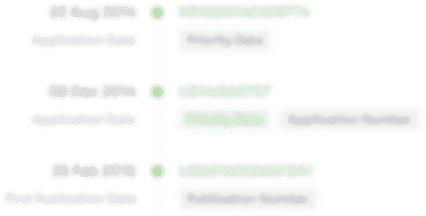
- Generate Ideas
- Intellectual Property
- Life Sciences
- Materials
- Tech Scout
- Unparalleled Data Quality
- Higher Quality Content
- 60% Fewer Hallucinations
Browse by: Latest US Patents, China's latest patents, Technical Efficacy Thesaurus, Application Domain, Technology Topic, Popular Technical Reports.
© 2025 PatSnap. All rights reserved.Legal|Privacy policy|Modern Slavery Act Transparency Statement|Sitemap|About US| Contact US: help@patsnap.com