Federal learning method and device
A learning method and federated technology, applied in the field of machine learning, can solve problems such as poisoning attacks and evil, and achieve the effect of preventing adverse effects
- Summary
- Abstract
- Description
- Claims
- Application Information
AI Technical Summary
Problems solved by technology
Method used
Image
Examples
Embodiment 1
[0073] Before federated learning starts, the server initializes global model parameters, aggregates weights, defines loss functions, initializes training rounds and verification rounds, and sends the initialized model parameters to each client for a certain round of federated training and verification .
[0074] During training rounds, such as figure 2 As shown, the execution steps are as follows:
[0075] Step 1: The server selects multiple clients participating in the training in this round, marks them as training clients, and sends the federated global model to the training clients.
[0076] Step 2: Train the client to train the model with local data, update the model parameters and gradients, and send the trained local model parameters (or gradients, that is, update parameters) to the server.
[0077] Step 3: The server aggregates the local model parameters (or gradients) uploaded by each training client to obtain the updated federated global model for this round.
[0...
Embodiment 2
[0084] The application scenario of this embodiment can be: in the background of federated learning, there are several participants' clients and a server's server, and the server jointly maintains a global model by aggregating the model parameters (or gradients) provided by the participants , in actual scenarios, it is impossible to guarantee that the models uploaded by all participants are reliable, so a node credibility verification method based on client-side interactive verification is proposed.
[0085] Specifically, it can be used when there is a server and clients of K parties , the kth client local storage has data samples , Represented as the i-th training sample of the k-th client, the server initializes the model parameters , defining the loss function as . Perform federated learning for R communication rounds Until the model converges, there are T training rounds whose index is and E verification round indices are ,and , the distribution initi...
Embodiment 3
[0121] In the privacy enhancement framework of homomorphic encryption, the client of the participant and the server of the server jointly establish the public key pk and private key sk based on the homomorphic encryption scheme. The private key sk is kept secret from the cloud server, but known to all study participants. Each participant will establish a TLS / SSL secure channel different from each other to communicate and protect the integrity of the homomorphic ciphertext. Among them, use Represents data encrypted with a public key.
[0122] In the training round, the specific execution steps are as follows:
[0123] Step 1: The server dispatches the model. In the current round r, the server sends the global model of this round For the training client participating in the next round of training .
[0124] Step 2: The client trains, updates, encrypts, and uploads the local model. training client Decrypt with private key sk get , and then use the local data tra...
PUM
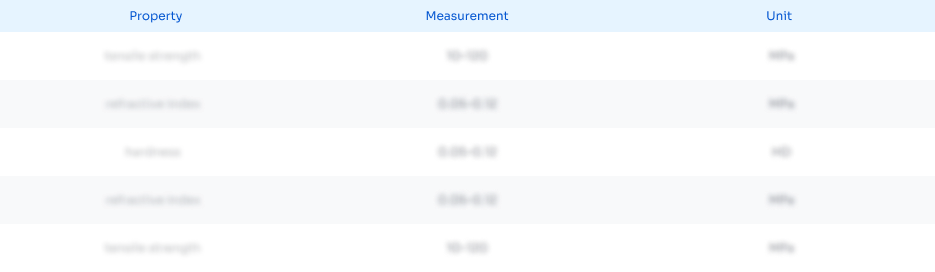
Abstract
Description
Claims
Application Information
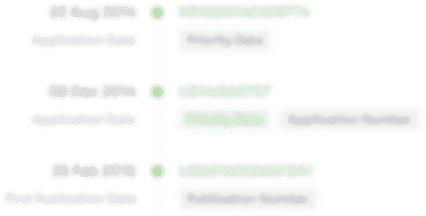
- R&D Engineer
- R&D Manager
- IP Professional
- Industry Leading Data Capabilities
- Powerful AI technology
- Patent DNA Extraction
Browse by: Latest US Patents, China's latest patents, Technical Efficacy Thesaurus, Application Domain, Technology Topic, Popular Technical Reports.
© 2024 PatSnap. All rights reserved.Legal|Privacy policy|Modern Slavery Act Transparency Statement|Sitemap|About US| Contact US: help@patsnap.com