Multi-task Chinese entity naming recognition method
An entity naming and recognition method technology, applied in neural learning methods, character and pattern recognition, instruments, etc., can solve problems such as difficulty in dividing entity boundaries, wrong word segmentation outside the vocabulary, and unsatisfactory BILSTM feature extractor effect. time consuming effect
- Summary
- Abstract
- Description
- Claims
- Application Information
AI Technical Summary
Problems solved by technology
Method used
Image
Examples
Embodiment Construction
[0067] Below in conjunction with embodiment the specific embodiment of the present invention is described in further detail:
[0068] Build the model and train it:
[0069] Divide the experimental data set into training set, validation set, and test set, and use BIO labeling rules for entity labeling. The tags used are Begin-named entity, In-named entity, and Out. When the named entity is described by a word, it is marked as Begin-named entity. When the named entity is described by a word, the word beginning with Begin-named entity is marked , other words are marked as In-named entity, and words that are not named entities are marked as Out. Then build the BERT-BI-BiLSTM-CRF network structure, which includes a bidirectional encoder\decoder, a double-layer long-short-term memory network layer, an attention network, a hidden layer, and a conditional random field layer. The encoder, decoder, double-layer long-term short-term memory network layer and conditional random field lay...
PUM
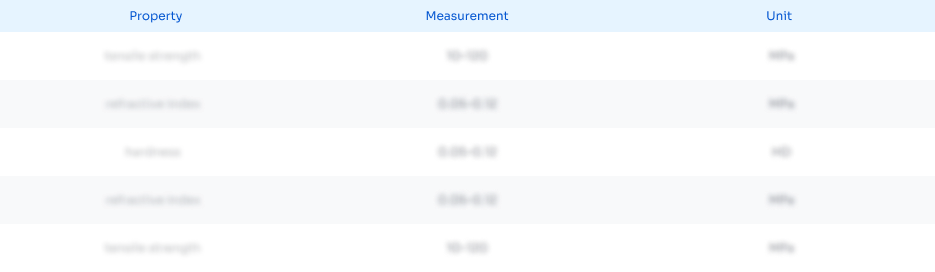
Abstract
Description
Claims
Application Information
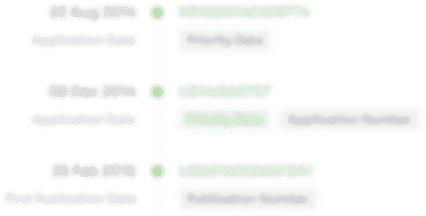
- R&D Engineer
- R&D Manager
- IP Professional
- Industry Leading Data Capabilities
- Powerful AI technology
- Patent DNA Extraction
Browse by: Latest US Patents, China's latest patents, Technical Efficacy Thesaurus, Application Domain, Technology Topic, Popular Technical Reports.
© 2024 PatSnap. All rights reserved.Legal|Privacy policy|Modern Slavery Act Transparency Statement|Sitemap|About US| Contact US: help@patsnap.com