Target detection method and device based on multi-gating hybrid expert model
A hybrid expert and target detection technology, applied in neural learning methods, biological neural network models, character and pattern recognition, etc., can solve problems such as damage to target detection accuracy, rough joint learning strategies, etc., to improve detection accuracy, avoid The effect of negatively affecting each other and improving efficiency
- Summary
- Abstract
- Description
- Claims
- Application Information
AI Technical Summary
Problems solved by technology
Method used
Image
Examples
Embodiment 1
[0071] Embodiment 1 provides an object detection method based on a multi-gated mixed expert model, which solves the problem of multi-task joint learning of object classification and bounding box regression, thereby improving the accuracy of the object detection method. The overall framework is as figure 2 As shown, first input the image into the deep convolutional neural network to obtain multi-scale features, then input the multi-scale features into the feature pyramid network to obtain the fused multi-scale features, further, input the multi-scale features into the The area recommendation network and the area pooling layer process to obtain the candidate area features, and finally input the candidate area features into the multi-gated mixed expert model to obtain the category and location of the object. Its process is as follows figure 1 Shown, specifically, described method comprises the following steps:
[0072] S1: Obtain the target feature map of the region where the ...
Embodiment 2
[0135] Image 6 It is a schematic structural diagram of a target detection device based on a multi-gated mixed expert model provided according to a specific embodiment of this patent. like Image 6 As shown, the system includes: an acquisition module 60 , a first processing module 61 , a second processing module 62 , and a determination module 63 .
[0136] Obtaining module 60, acquiring the target feature map and the potential target frame of the region where the potential target is located in the image;
[0137] The first processing module 61 processes the target feature map using an expert model, and outputs the target classification subtask result and the bounding box regression parameter subtask result corresponding to the target feature map; the quantity of the expert model includes multiple Each expert model outputs its target classification subtask results and bounding box regression parameter subtask results respectively;
[0138] The second processing module 62 pr...
PUM
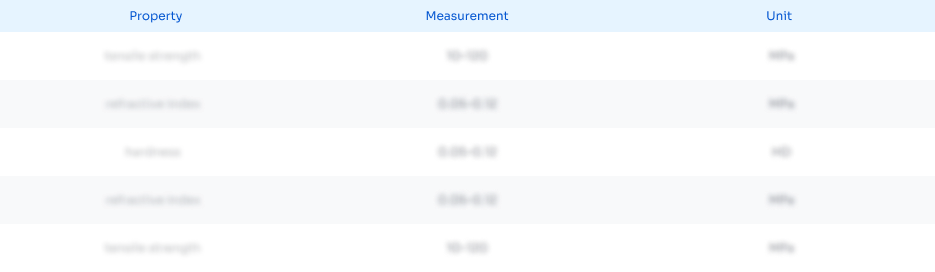
Abstract
Description
Claims
Application Information
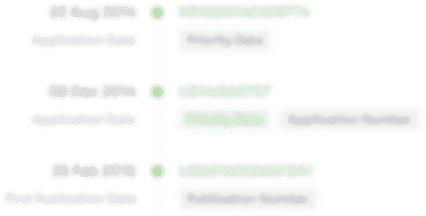
- R&D Engineer
- R&D Manager
- IP Professional
- Industry Leading Data Capabilities
- Powerful AI technology
- Patent DNA Extraction
Browse by: Latest US Patents, China's latest patents, Technical Efficacy Thesaurus, Application Domain, Technology Topic.
© 2024 PatSnap. All rights reserved.Legal|Privacy policy|Modern Slavery Act Transparency Statement|Sitemap