Classification tree construction method and data processing method based on classification tree
A construction method and a classification tree technology, applied in the computer field, to achieve the effect of improving performance
- Summary
- Abstract
- Description
- Claims
- Application Information
AI Technical Summary
Problems solved by technology
Method used
Image
Examples
Embodiment 1
[0043] A classification tree construction method, comprising the following steps:
[0044] 1) Initialize the classification tree, the specific content is: collect sample data, generate a root node, attribute all samples to the root node, take the mode of the sample set class mark as the class mark of the root node, and initialize the root node at the same time is the current only leaf node.
[0045] 2) Traverse the nodes and judge whether they are divisible;
[0046] 2-1 Traversing all nodes, looking for the leaf nodes in the deepest layer of the current classification tree;
[0047] 2-2 Calculate the Gini index of a node, assuming that the sample subset contained in the node is D, then the calculation method of the Gini index is:
[0048]
[0049] In the formula, K is the number of sample categories contained in D, p k It is the proportion of samples of the kth class.
[0050] 2-3 Setting Threshold Th min , Th min The minimum number of samples required for node splitti...
Embodiment 2
[0072] The risk of heart disease of the subject is predicted based on the classification tree constructed by the method of the first embodiment by using the physical measurement indexes related to the human body.
[0073] Construct a heart disease data set. The characteristics of the data set include: age, gender, type of angina pectoris, resting blood pressure, cholesterol, fasting blood sugar, and electrocardiogram; the data label is whether it is heart disease.
[0074] Age, resting blood pressure, cholesterol, and fasting blood glucose in the data set are continuous feature attributes, gender and angina pectoris type are discrete feature attributes, and electrocardiogram is a vector feature attribute.
[0075] By training a classification tree with the constructed data set, it is possible to predict whether a new case has a heart disease risk.
Embodiment 3
[0077] The classification tree constructed based on the method of Embodiment 1 uses the characteristic attributes of vertebrates to judge the animal type.
[0078] Construct a vertebrate data set. The characteristics of the data set include: body temperature, epidermis coverage, whether it is viviparous, whether it is aquatic, whether it is flying, whether it has legs, whether it hibernates, whether it is hibernating, body length, and gene sequence; the data labels are animals, including mammals, reptiles species, fish, amphibians, birds.
[0079] Body temperature and body length in the data set are continuous feature attributes, epidermis coverage, viviparous, aquatic, flying, legged, and hibernating are discrete feature attributes, and gene sequences are vector feature attributes.
[0080] The type of newly discovered animal species can be judged by training the classification tree with the constructed data set.
PUM
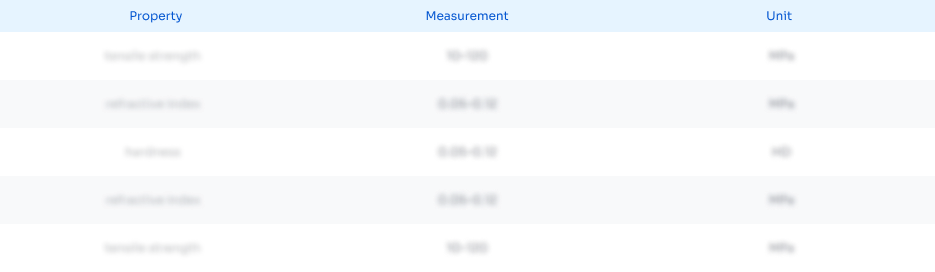
Abstract
Description
Claims
Application Information
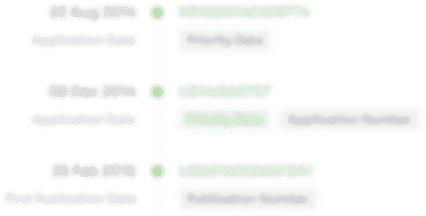
- R&D Engineer
- R&D Manager
- IP Professional
- Industry Leading Data Capabilities
- Powerful AI technology
- Patent DNA Extraction
Browse by: Latest US Patents, China's latest patents, Technical Efficacy Thesaurus, Application Domain, Technology Topic.
© 2024 PatSnap. All rights reserved.Legal|Privacy policy|Modern Slavery Act Transparency Statement|Sitemap