Semantic segmentation-based unstructured field road scene recognition method and device
A semantic segmentation and unstructured technology, applied in the field of agricultural field road scene recognition, can solve the problems of insufficient consideration of image context information, low segmentation accuracy of complex scenes, and low utilization of global features, so as to improve feature utilization efficiency and The effect of predicting consistency, maintaining continuity and integrity, and balancing accuracy and speed
- Summary
- Abstract
- Description
- Claims
- Application Information
AI Technical Summary
Problems solved by technology
Method used
Image
Examples
Embodiment Construction
[0039] The specific embodiments of the invention will be further described below in conjunction with the accompanying drawings. The following examples are only used to more clearly illustrate the technical solutions of the present invention, and cannot be used to limit the protection scope of the present invention.
[0040] figure 1 A schematic flow chart of a semantic segmentation-based unstructured field road scene recognition method provided by an embodiment of the present invention, as shown in figure 1 As shown, the method includes the following steps:
[0041] 101. Obtain a data set constructed from unstructured field road scene images, and perform semantic annotation on the image data set;
[0042] 102. Perform data amplification on the labeled data set, and divide the amplified data into a training set, a verification set, and a test set;
[0043] 103. Construct a semantic segmentation model based on the Keras deep learning framework, incorporate hybrid expansion con...
PUM
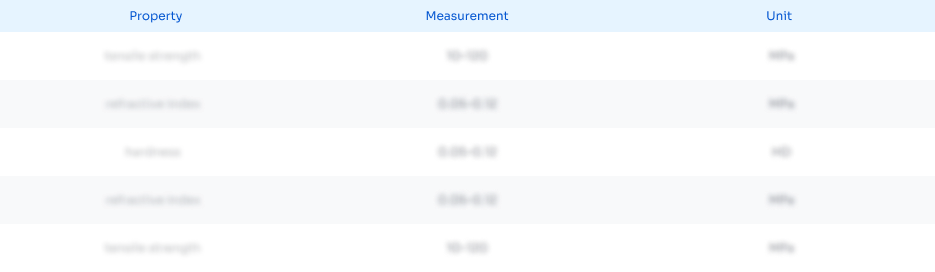
Abstract
Description
Claims
Application Information
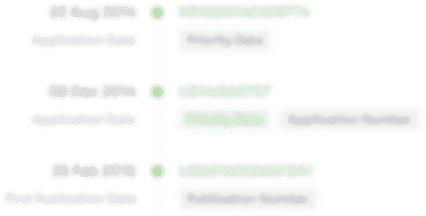
- R&D
- Intellectual Property
- Life Sciences
- Materials
- Tech Scout
- Unparalleled Data Quality
- Higher Quality Content
- 60% Fewer Hallucinations
Browse by: Latest US Patents, China's latest patents, Technical Efficacy Thesaurus, Application Domain, Technology Topic, Popular Technical Reports.
© 2025 PatSnap. All rights reserved.Legal|Privacy policy|Modern Slavery Act Transparency Statement|Sitemap|About US| Contact US: help@patsnap.com