Attention-optimized deep coding and decoding defogging generative adversarial network
An attention, encoding and decoding technology, applied in the field of image processing, can solve the problems of low complexity, difficult to obtain dehazing data sets, error superposition, etc., to achieve the effect of recovering information loss, good training effect, and strong robustness
- Summary
- Abstract
- Description
- Claims
- Application Information
AI Technical Summary
Problems solved by technology
Method used
Image
Examples
Embodiment Construction
[0021] Below in conjunction with accompanying drawing and specific embodiment the present invention is further described:
[0022] 1 Overview
[0023] The attention-optimized deep codec defogging generative adversarial network of the present invention is a dehazing generative adversarial network based on a codec architecture. The generative confrontation network of the present invention adopts an encoder with a four-layer downsampling structure to fully extract semantic information lost due to fog so as to restore a clear image. At the same time, in the decoder network, an attention mechanism is introduced to adaptively assign weights to different pixels and channels, so as to deal with the unevenly distributed fog. Finally, the framework of generative confrontation network enables the model to achieve better training effect on small sample data sets.
[0024] The experimental results show that the dehazing network of the present invention can not only effectively remove the...
PUM
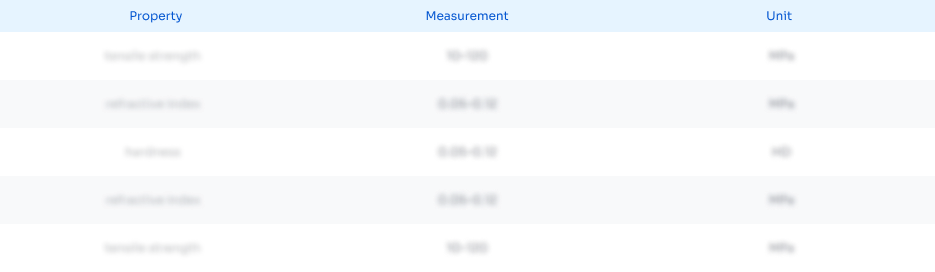
Abstract
Description
Claims
Application Information
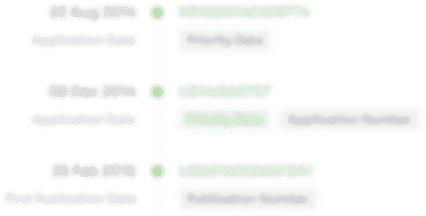
- R&D
- Intellectual Property
- Life Sciences
- Materials
- Tech Scout
- Unparalleled Data Quality
- Higher Quality Content
- 60% Fewer Hallucinations
Browse by: Latest US Patents, China's latest patents, Technical Efficacy Thesaurus, Application Domain, Technology Topic, Popular Technical Reports.
© 2025 PatSnap. All rights reserved.Legal|Privacy policy|Modern Slavery Act Transparency Statement|Sitemap|About US| Contact US: help@patsnap.com