LDCT image super-resolution enhancement method and device based on residual convolutional neural network
A convolutional neural network, CT image technology, applied in image enhancement, neural learning methods, biological neural network models, etc., can solve the problems of high cost, inability to adapt, slow imaging speed, etc., achieve non-destructive high-resolution detection, improve Image structure similarity and the effect of improving image signal-to-noise ratio
- Summary
- Abstract
- Description
- Claims
- Application Information
AI Technical Summary
Problems solved by technology
Method used
Image
Examples
Embodiment Construction
[0054] The technical solutions of the present invention will be further described below in conjunction with the accompanying drawings and examples of implementation.
[0055] as attached figure 1 Shown is a kind of flow chart diagram of the present invention, comprises the following steps:
[0056] Step 1) Make training set and test set;
[0057] Step 2) LDCT initial image preprocessing;
[0058] Step 3) Judging whether to train, if yes, go to step 4), if not, go to step 8);
[0059] Step 4) Improve the mixed cascade task U-Net for feature extraction;
[0060] Step 5) Error calculation;
[0061] Step 6) Error backpropagation;
[0062] Step 7) Judging whether the error meets the requirements, if yes, go to step 8), if not, return to step 4);
[0063] Step 8) output image super-resolution model;
[0064] Step 9) generating a super-resolution CT image;
[0065] Step 10) End.
[0066] Among them, the steps of making training set and test set are as follows:
[0067] Step...
PUM
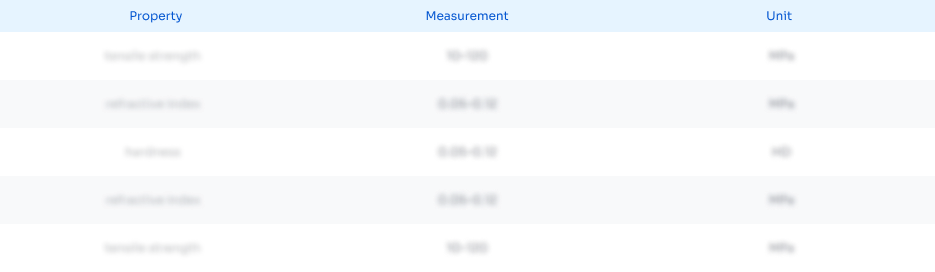
Abstract
Description
Claims
Application Information
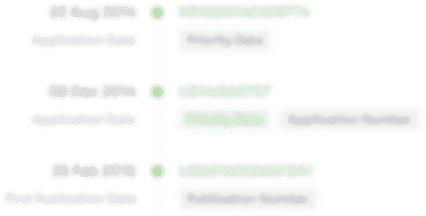
- R&D Engineer
- R&D Manager
- IP Professional
- Industry Leading Data Capabilities
- Powerful AI technology
- Patent DNA Extraction
Browse by: Latest US Patents, China's latest patents, Technical Efficacy Thesaurus, Application Domain, Technology Topic, Popular Technical Reports.
© 2024 PatSnap. All rights reserved.Legal|Privacy policy|Modern Slavery Act Transparency Statement|Sitemap|About US| Contact US: help@patsnap.com