Image Generation Method for Irregular Hole Morphology Defects
A technology for image generation and irregularity, which is applied in image analysis, image data processing, optical test flaws/defects, etc. It can solve the problems of hole shape defects that have not been touched by anyone, and are different, so as to shorten the online cycle and model iteration cycle. Reduce the iteration cycle and ensure the effect of stability
- Summary
- Abstract
- Description
- Claims
- Application Information
AI Technical Summary
Problems solved by technology
Method used
Image
Examples
Embodiment 1
[0046] 1. Randomly select a normal image from the project dataset as the background image generated by the defect, such as image 3 shown.
[0047] 2. Randomly sample the coordinate points in the set area as the center of the circle, and use the distance r as the radius to define a circle as the area where the hole shape defects are generated, such as Figure 4 shown. Randomly select n pixel coordinate points ... in the picture as the bottom area contour points, where the positions of each point are respectively. ....., as in Figure 5 shown.
[0048] 3. Fit the bottom region contour points randomly selected in the previous step with the Bezier curve method, and randomly select the shape factor K of the curve, where K belongs to [0.2, 0.6]. The current K value is 0.3, and you get a value such as Image 6 The closed irregular circle in , the circularity of its contour area is greater than 0.5.
[0049] 4. The closed irregular circle generated in step 3 is defined as the b...
PUM
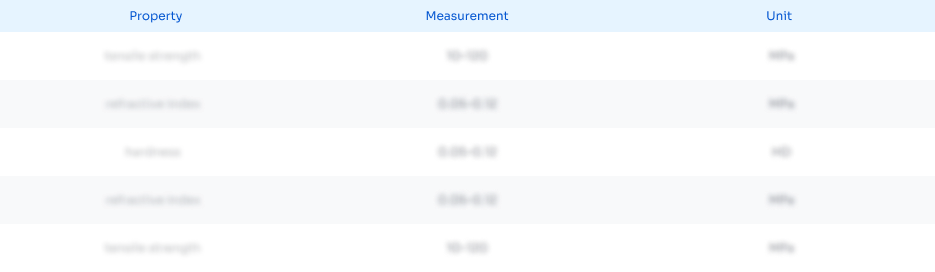
Abstract
Description
Claims
Application Information
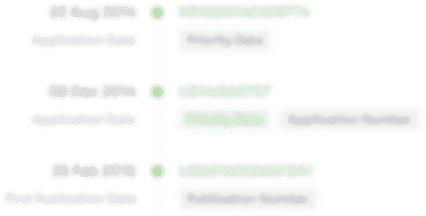
- R&D Engineer
- R&D Manager
- IP Professional
- Industry Leading Data Capabilities
- Powerful AI technology
- Patent DNA Extraction
Browse by: Latest US Patents, China's latest patents, Technical Efficacy Thesaurus, Application Domain, Technology Topic, Popular Technical Reports.
© 2024 PatSnap. All rights reserved.Legal|Privacy policy|Modern Slavery Act Transparency Statement|Sitemap|About US| Contact US: help@patsnap.com