Remote sensing multispectral data semi-supervised labeling method based on self-learning
A multi-spectral, self-learning technology, applied in the field of data labeling, can solve the problems of heavy manual labeling workload, limited target types, difficult area data labeling, etc., and achieve the effects of rich categories, fast classification speed, and improved labeling accuracy
- Summary
- Abstract
- Description
- Claims
- Application Information
AI Technical Summary
Problems solved by technology
Method used
Image
Examples
Embodiment Construction
[0047]A method of self-learning-based remote sensing multi-spectral data semi-supervision labeling method, through various categories, manually labeled a small amount of seed points, based on self-learning algorithm and abnormal point detection algorithm, the classification of non-label data, mainly including four processes Sub-cycle iteration: 1) Get tag data; 2) Tag data pretreatment; 3) Based on the self-learning algorithm iteration based on tape tag data; use iterative after algorithm to classify the label data, acquire the updated band tag data; 4 ) Remove anomalum point in tag data.
[0048] The present invention can realize the data label of any category, the amount of manual label data is low, and the automatic annotation process can be completed on the basis of less labeling data, and the way to learn from the way, and the label is fast, avoiding artificial marking. The experience of data is different.
[0049] The present invention will be further described in detail bel...
PUM
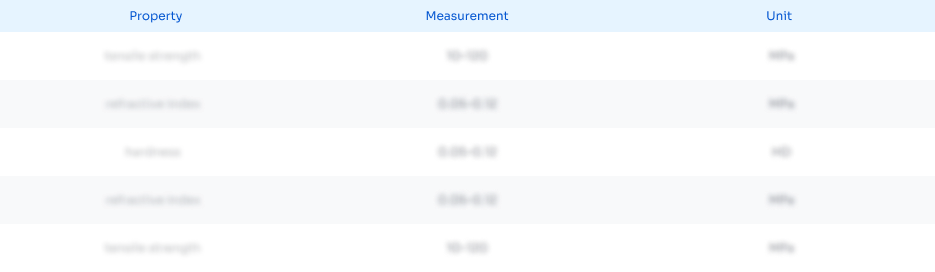
Abstract
Description
Claims
Application Information
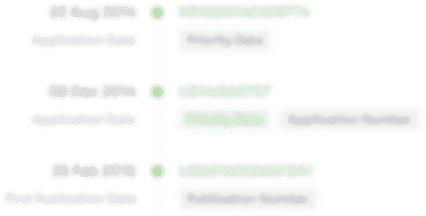
- R&D
- Intellectual Property
- Life Sciences
- Materials
- Tech Scout
- Unparalleled Data Quality
- Higher Quality Content
- 60% Fewer Hallucinations
Browse by: Latest US Patents, China's latest patents, Technical Efficacy Thesaurus, Application Domain, Technology Topic, Popular Technical Reports.
© 2025 PatSnap. All rights reserved.Legal|Privacy policy|Modern Slavery Act Transparency Statement|Sitemap|About US| Contact US: help@patsnap.com