Central air conditioner control method based on multi-agent deep reinforcement learning
A reinforcement learning, multi-agent technology, applied in neural learning methods, control input involving air characteristics, space heating and ventilation control input, etc., can solve problems such as large action space, long training time, and lack of robustness. , to achieve the effect of reducing the total energy consumption of the building, improving the service life and reducing the failure rate
- Summary
- Abstract
- Description
- Claims
- Application Information
AI Technical Summary
Problems solved by technology
Method used
Image
Examples
Embodiment Construction
[0044] Below in conjunction with specific embodiment, content of the present invention is described in further detail:
[0045] combine Figure 1 to Figure 5 , this embodiment is a central air-conditioning control method based on multi-agent deep reinforcement learning. According to the current indoor demand cooling load and outdoor wet-bulb temperature, the start-stop status and Model-free optimal control of working parameters, including cooling machine running sequence control, and intelligent body optimal control of cooling water pump and cooling tower fan operating frequency.
[0046] Such as figure 1 As shown, the intercooler, cooling water pump and cooling water tower of the central air-conditioning system are connected in sequence and arranged in groups, such as figure 2 As shown, the sequence control of the chiller is realized by a sequence controller, and the intelligent body optimal control of the working frequency of the cooling water pump and the cooling tower f...
PUM
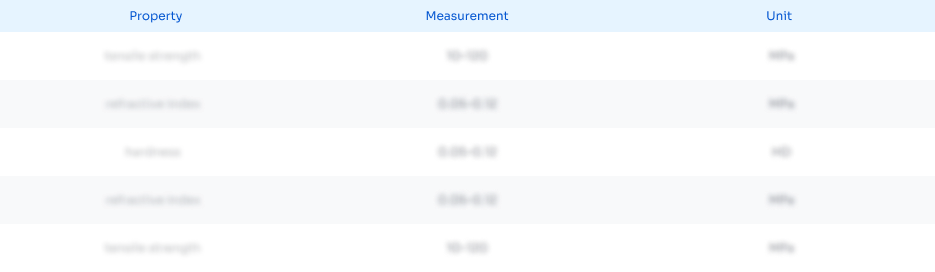
Abstract
Description
Claims
Application Information
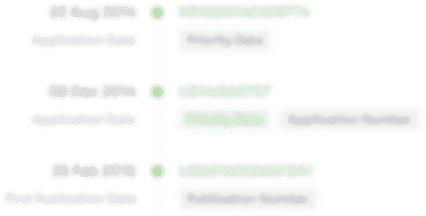
- R&D Engineer
- R&D Manager
- IP Professional
- Industry Leading Data Capabilities
- Powerful AI technology
- Patent DNA Extraction
Browse by: Latest US Patents, China's latest patents, Technical Efficacy Thesaurus, Application Domain, Technology Topic.
© 2024 PatSnap. All rights reserved.Legal|Privacy policy|Modern Slavery Act Transparency Statement|Sitemap