Cross-regional cross-score collaborative filtering recommendation method and system
A collaborative filtering recommendation and cross-regional technology, applied in the field of cross-regional cross-scoring collaborative filtering recommendation method and system, can solve the problems of sparseness and inaccurate scoring prediction in sparsely scored regions, so as to improve performance, avoid negative transfer, and improve accuracy Effect
- Summary
- Abstract
- Description
- Claims
- Application Information
AI Technical Summary
Problems solved by technology
Method used
Image
Examples
Embodiment 1
[0042] like figure 1 As shown, this embodiment provides a cross-region and cross-scoring collaborative filtering recommendation method, including the following steps:
[0043] Step 1: Obtain the user-item rating data of the target domain and the source domain;
[0044] Step 2: Compose the user-item scoring data of the target domain and the source domain into a target domain scoring matrix and a source domain scoring matrix;
[0045] Step 3: sort the users and items in the scoring matrix of the target domain according to the number of ratings; divide all users into active users and inactive users according to the threshold, and divide all items into popular items and non-popular items;
[0046] Step 4: Based on the latent semantic Funk-SVD model, perform matrix decomposition on the scoring matrices of the target domain and the source domain, respectively, and extract hidden vectors of users and items in the target domain and source domain;
[0047] Step 5: For active users an...
Embodiment 2
[0124] This embodiment provides a cross-region and cross-scoring collaborative filtering recommendation system, including:
[0125] The data preprocessing module is configured to: obtain the user-item scoring data of the target domain and the source domain, and obtain the target domain scoring matrix and the source domain scoring matrix after preprocessing;
[0126] Divide all users in the target domain scoring matrix and source domain scoring matrix into active users and inactive users, and divide all projects into popular projects and non-popular projects;
[0127] The feature extraction module is configured to: decompose the target domain scoring matrix and the source domain scoring matrix based on the latent semantic model, and extract user latent vectors and item latent vectors in the target domain and the source domain;
[0128] For active users and popular projects, based on the trained deep regression network, learn the mapping relationship between the target domain and ...
Embodiment 3
[0132] This embodiment provides a computer-readable storage medium, on which a computer program is stored. When the program is executed by a processor, the steps in the cross-region and cross-scoring collaborative filtering recommendation method as described above are implemented.
PUM
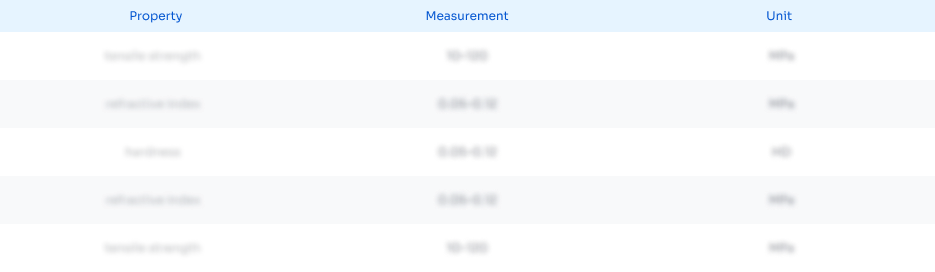
Abstract
Description
Claims
Application Information
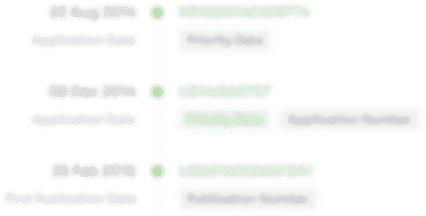
- R&D
- Intellectual Property
- Life Sciences
- Materials
- Tech Scout
- Unparalleled Data Quality
- Higher Quality Content
- 60% Fewer Hallucinations
Browse by: Latest US Patents, China's latest patents, Technical Efficacy Thesaurus, Application Domain, Technology Topic, Popular Technical Reports.
© 2025 PatSnap. All rights reserved.Legal|Privacy policy|Modern Slavery Act Transparency Statement|Sitemap|About US| Contact US: help@patsnap.com